Using business intelligence to improve supply chain management efficiency is no longer a futuristic concept; it’s a crucial strategy for businesses aiming to thrive in today’s dynamic market. This deep dive explores how leveraging data-driven insights can revolutionize your supply chain, from optimizing inventory to predicting demand and strengthening supplier relationships. Get ready to unlock the power of BI and transform your logistics game!
Inefficient supply chains lead to lost revenue, stockouts, and unhappy customers. Business intelligence (BI), the process of transforming data into actionable insights, offers a powerful solution. By integrating data from various sources – sales figures, inventory levels, transportation costs, and more – BI tools provide a holistic view of your supply chain, revealing bottlenecks and opportunities for improvement. This allows for data-driven decision-making, leading to optimized processes and significant cost savings.
Introduction
In today’s hyper-competitive business landscape, optimizing supply chain management is paramount. Business intelligence (BI) plays a crucial role in achieving this efficiency. Understanding the relationship between these two concepts is key to unlocking significant operational improvements and gaining a competitive edge.Business intelligence and efficient supply chain management are intrinsically linked. BI involves the strategies and technologies used to collect, integrate, analyze, and present business information.
This data-driven approach provides valuable insights that inform better decision-making across all aspects of an organization. Supply chain management, on the other hand, encompasses the planning, execution, and control of all activities involved in procuring raw materials, transforming them into finished goods, and delivering them to the end customer. The seamless integration of BI into supply chain management allows for real-time visibility, predictive analytics, and proactive problem-solving, ultimately leading to a more efficient and responsive supply chain.
Inefficient Supply Chains: Business Impacts
Inefficient supply chains can significantly harm a business’s bottom line and reputation. Delays in delivery, stockouts, excess inventory, and poor quality control are all common consequences of poorly managed supply chains. For example, a company experiencing frequent stockouts might lose sales and customers to competitors who can consistently meet demand. Conversely, holding excessive inventory ties up capital and increases storage costs, impacting profitability.
A significant disruption, such as a natural disaster affecting a key supplier, can bring entire operations to a standstill, causing substantial financial losses and reputational damage. Consider the impact of the 2011 Tohoku earthquake and tsunami on the global automotive industry; the disruption to the supply of parts from Japan caused widespread production halts and significant financial losses for many manufacturers.
These disruptions highlight the critical need for robust and responsive supply chains capable of withstanding unforeseen events. The ability to anticipate and mitigate such risks is where BI plays a crucial role.
Data Sources for Supply Chain BI
Harnessing the power of Business Intelligence (BI) in supply chain management hinges on access to reliable and comprehensive data. Without accurate data reflecting the intricate network of processes, from procurement to delivery, effective optimization is impossible. This section delves into the key data sources fueling successful supply chain BI initiatives.
Effective supply chain management relies on a multitude of data points, meticulously collected and analyzed to reveal crucial insights. These insights inform strategic decisions, leading to enhanced efficiency and profitability. The data itself comes from various sources, each contributing a vital piece to the puzzle.
Key Data Sources and Their Types
The following table categorizes key data sources used in supply chain BI, detailing the types of data collected, their frequency, and their overall value to the organization.
Data Source | Data Type | Frequency | Value |
---|---|---|---|
Enterprise Resource Planning (ERP) Systems | Inventory levels, production schedules, order processing data, material costs, sales data | Real-time or near real-time, depending on system configuration | Provides a holistic view of internal operations, enabling proactive inventory management and production planning. |
Customer Relationship Management (CRM) Systems | Customer orders, sales forecasts, customer demographics, delivery preferences | Real-time or near real-time, depending on system configuration | Offers insights into customer demand, enabling accurate forecasting and optimized inventory levels. Helps anticipate potential disruptions. |
Transportation Management Systems (TMS) | Shipment tracking data, transportation costs, delivery times, carrier performance metrics | Real-time or near real-time, depending on system configuration | Facilitates efficient route planning, cost optimization, and improved delivery performance. Identifies potential bottlenecks in the logistics process. |
Warehouse Management Systems (WMS) | Inventory levels, location data, stock movements, picking and packing times | Real-time or near real-time, depending on system configuration | Provides granular visibility into warehouse operations, enabling optimization of storage space, order fulfillment processes, and labor utilization. |
Supplier Relationship Management (SRM) Systems | Supplier performance data, lead times, material quality, delivery schedules | Regular intervals, often weekly or monthly | Enables strategic supplier selection, performance monitoring, and risk mitigation. Improves collaboration and communication with suppliers. |
Point of Sale (POS) Systems | Sales transactions, product demand, pricing information | Real-time | Provides immediate feedback on product performance and market trends, facilitating dynamic pricing strategies and inventory adjustments. |
Social Media and Market Research | Customer sentiment, brand perception, market trends | Continuous monitoring | Offers insights into customer preferences and market dynamics, informing product development and marketing strategies. Can identify potential supply chain disruptions. |
BI Tools and Technologies for Supply Chain Optimization
Harnessing the power of Business Intelligence (BI) is crucial for modern supply chain management. Effective BI tools provide the analytical capabilities needed to uncover hidden patterns, predict future trends, and optimize various aspects of the supply chain, from procurement to delivery. By leveraging data-driven insights, businesses can significantly improve efficiency, reduce costs, and enhance overall responsiveness.
Overview of BI Tools for Supply Chain Management
Several BI tools and technologies are specifically designed or adaptable for optimizing supply chain operations. These range from sophisticated enterprise resource planning (ERP) systems with embedded analytics to specialized supply chain management (SCM) software and cloud-based platforms offering advanced analytics and visualization capabilities. The choice of the right tool depends heavily on factors like the size and complexity of the supply chain, budget constraints, and the specific analytical needs of the organization.
Comparison of Three Leading BI Tools
Three prominent BI tools frequently employed in supply chain optimization are Tableau, Power BI, and SAP Analytics Cloud. Each offers unique strengths and weaknesses.Tableau is known for its intuitive drag-and-drop interface and powerful visualization capabilities, making it relatively easy to create compelling dashboards and reports even for users with limited technical expertise. However, its cost can be a significant barrier for smaller businesses, and advanced analytical functionalities might require specialized training.Power BI, Microsoft’s offering, integrates seamlessly with other Microsoft products, making it a strong choice for organizations already heavily invested in the Microsoft ecosystem.
Its cost-effectiveness and strong data connectivity are major advantages. However, its customization options can be less extensive compared to Tableau, and complex data modeling might require more technical skills.SAP Analytics Cloud, a cloud-based solution, offers a comprehensive suite of analytical tools integrated with SAP’s ERP systems. This tight integration is a significant advantage for businesses using SAP, streamlining data access and analysis.
However, its reliance on the SAP ecosystem limits its flexibility for organizations using other ERP systems, and the pricing model can be complex.
Comparative Analysis of BI Tools for Supply Chain Management
Feature | Tableau | Power BI | SAP Analytics Cloud |
---|---|---|---|
Data Connectivity | Wide range of connectors | Strong integration with Microsoft products | Strong integration with SAP systems |
Visualization Capabilities | Excellent, highly customizable | Good, user-friendly | Good, integrated with SAP reporting |
Analytical Capabilities | Advanced, requires expertise for full utilization | Good, user-friendly for basic analysis | Comprehensive, integrated with SAP analytics |
Cost | High, tiered pricing | Relatively low, tiered pricing | Moderate to high, dependent on features and users |
Suitability for Small Businesses | Limited due to cost | Good, cost-effective | Limited unless already using SAP systems |
Suitability for Large Enterprises | Excellent, scalable | Excellent, scalable | Excellent, especially for SAP users |
Improving Forecasting Accuracy with BI
Business intelligence (BI) significantly enhances supply chain forecasting accuracy by leveraging vast datasets and advanced analytical capabilities. This leads to more informed decisions, optimized inventory levels, and ultimately, a more efficient and profitable supply chain. By integrating data from various sources, BI tools provide a holistic view of demand patterns, enabling businesses to predict future needs with greater precision.BI improves demand forecasting accuracy by providing a more comprehensive and nuanced understanding of market dynamics.
Traditional forecasting methods often rely on limited data points and simpler models, making them susceptible to inaccuracies. BI, however, allows businesses to analyze historical sales data, market trends, economic indicators, social media sentiment, and even weather patterns – all factors that can influence demand. This multifaceted approach leads to more accurate predictions, reducing the risk of miscalculations.
Minimizing Stockouts and Overstocking through Improved Forecasting
Accurate forecasting, powered by BI, directly impacts inventory management. By predicting demand more precisely, companies can optimize their stock levels, avoiding both stockouts and overstocking. Stockouts lead to lost sales, dissatisfied customers, and damage to brand reputation. Conversely, overstocking ties up capital in excess inventory, increasing storage costs and potentially leading to obsolescence or spoilage. BI’s predictive capabilities help strike the optimal balance, ensuring sufficient stock to meet demand without excessive surplus.
For example, a retailer using BI to analyze sales data and seasonal trends could accurately predict a surge in demand for winter coats during November and December. This allows them to procure and stock the appropriate quantities, avoiding both stockouts during peak season and excessive inventory during the off-season.
Examples of Forecasting Methods Enhanced by BI Tools
Several forecasting methods benefit significantly from the capabilities of BI tools. Traditional methods like moving averages and exponential smoothing can be enhanced by incorporating additional data sources and advanced analytical techniques. BI platforms can automate the process of data cleaning, transformation, and model building, making forecasting more efficient and less prone to human error. More sophisticated methods, such as machine learning algorithms, become readily accessible and manageable through BI’s user-friendly interfaces.
For instance, a manufacturer using a BI-powered forecasting system could implement a machine learning model to predict component demand based on historical data, production schedules, and even real-time sensor data from the factory floor. This leads to more accurate procurement planning and reduces the risk of production delays due to component shortages. Another example could involve a food retailer leveraging BI to analyze sales data, weather patterns, and social media trends to predict demand for perishable goods, optimizing ordering and reducing waste due to spoilage.
Enhancing Inventory Management
Real-time visibility into inventory levels is crucial for efficient supply chain management. Business intelligence (BI) provides the tools and insights to achieve this, optimizing stock levels, minimizing waste, and maximizing profitability. By leveraging data-driven insights, businesses can move from reactive inventory management to a proactive, predictive approach.Real-time inventory tracking significantly improves efficiency by providing an accurate, up-to-the-minute view of stock across the entire supply chain.
This eliminates the delays and inaccuracies associated with manual tracking methods, allowing for faster decision-making and improved responsiveness to changes in demand.
Optimized Inventory Levels Across the Supply Chain
BI tools analyze historical sales data, current demand forecasts, and lead times to calculate optimal inventory levels for each product at each stage of the supply chain. This prevents stockouts, which can lead to lost sales and dissatisfied customers, while simultaneously avoiding overstocking, which ties up capital and increases the risk of obsolescence or spoilage. Sophisticated BI systems can even incorporate external factors like weather patterns or economic indicators to refine these predictions and further optimize inventory levels.
For example, a retailer using BI might predict a surge in demand for winter coats based on weather forecasts and adjust their inventory accordingly, ensuring sufficient stock to meet the anticipated increase while avoiding excess inventory during warmer months.
Scenario: BI-Driven Inventory Management at a Clothing Retailer, Using business intelligence to improve supply chain management efficiency
Imagine a large clothing retailer struggling with inconsistent inventory levels. Before implementing BI, they experienced frequent stockouts of popular items and significant overstocking of less popular lines. This resulted in lost sales, increased storage costs, and higher markdown expenses. After implementing a BI system, the retailer gained real-time visibility into inventory across all its stores and warehouses.
The BI system analyzed historical sales data, seasonal trends, and promotional campaigns to predict demand accurately. This allowed them to optimize inventory levels, reducing stockouts by 20% and overstocking by 15%. The result was a significant increase in sales, a reduction in carrying costs, and improved customer satisfaction. The improved forecasting, driven by the BI system, allowed for more effective planning of production and procurement, leading to streamlined operations and a more responsive supply chain.
Furthermore, the real-time tracking capabilities allowed for quick identification and resolution of any inventory discrepancies, minimizing potential losses.
Optimizing Logistics and Transportation
Business intelligence (BI) is revolutionizing supply chain management, and nowhere is this more evident than in the optimization of logistics and transportation. By leveraging data-driven insights, companies can significantly reduce costs, improve delivery times, and enhance overall supply chain efficiency. This involves a sophisticated analysis of various factors, from route planning and carrier selection to real-time shipment tracking and exception management.BI tools provide the analytical power to transform raw logistics data into actionable intelligence, enabling businesses to make data-backed decisions that minimize operational inefficiencies and maximize profitability.
This translates to lower transportation costs, improved customer satisfaction through on-time deliveries, and a more resilient and responsive supply chain.
Route Optimization and Cost Reduction
BI systems analyze vast amounts of data – including historical shipping data, real-time traffic conditions, fuel prices, and even weather patterns – to identify the most efficient transportation routes. Algorithms analyze various factors to determine the optimal path, considering factors like distance, time, and cost. For instance, a company shipping goods across the country might use BI to compare different routes, considering potential traffic congestion at certain times of day or on specific days of the week.
By choosing the optimal route, they can minimize fuel consumption, reduce driver downtime, and ultimately lower shipping costs. This optimization extends beyond simply choosing the shortest route; it involves considering all relevant cost factors to arrive at the most economically viable option.
Real-Time Shipment Tracking and Management
Real-time visibility into the movement of goods is paramount in modern supply chain management. BI dashboards provide a centralized view of all shipments, displaying their current location, estimated time of arrival (ETA), and any potential delays or exceptions. This real-time tracking allows for proactive intervention. For example, if a shipment is delayed due to unforeseen circumstances like a traffic accident, the company can immediately reroute the shipment or notify the customer, minimizing disruption.
Optimizing supply chain management hinges on data-driven insights; Business Intelligence (BI) tools provide this crucial edge. To truly leverage BI’s power in manufacturing, consider integrating robust manufacturing software for seamless data flow. This integration allows for more accurate forecasting, streamlined inventory management, and ultimately, a more efficient and responsive supply chain. The result? Significant improvements in your bottom line thanks to better BI-powered decision-making.
Imagine a logistics company using a BI dashboard that shows a map with all their trucks, their current locations marked with real-time GPS data, and color-coded alerts indicating potential delays. This level of visibility allows for immediate response to unexpected events, ensuring on-time deliveries and satisfied customers.
A Step-by-Step Procedure for Logistics Optimization with BI
Implementing BI for logistics optimization involves a structured approach. Here’s a step-by-step procedure:
- Data Integration: Gather all relevant data from various sources, including transportation management systems (TMS), warehouse management systems (WMS), GPS tracking devices, and external data providers (e.g., weather services, traffic data). Ensure data quality and consistency.
- Data Cleaning and Transformation: Cleanse and standardize the collected data to ensure accuracy and reliability. This might involve handling missing values, correcting inconsistencies, and transforming data into a suitable format for analysis.
- Analytical Model Development: Develop analytical models using BI tools to identify patterns, trends, and insights within the data. This could involve using predictive analytics to forecast potential delays or optimize routes based on historical data and real-time conditions.
- Dashboard and Report Creation: Create interactive dashboards and reports to visualize key performance indicators (KPIs) and provide real-time insights into logistics operations. These dashboards should present information clearly and concisely, allowing for quick decision-making.
- Scenario Planning and What-If Analysis: Use BI tools to simulate different scenarios and evaluate the potential impact of various decisions. For example, you could model the impact of choosing different routes or carriers on overall transportation costs.
- Continuous Monitoring and Improvement: Continuously monitor logistics performance using BI dashboards and reports, and use the insights gained to identify areas for improvement and optimize processes over time.
Improving Supplier Relationships
Business intelligence (BI) is no longer just a tool for internal optimization; it’s a powerful engine for strengthening external partnerships, particularly with suppliers. By leveraging data-driven insights, companies can foster deeper collaboration, improve communication, and ultimately build more resilient and efficient supply chains. This leads to better product quality, reduced costs, and enhanced overall performance.BI fosters better collaboration with suppliers by providing a shared platform for data visualization and analysis.
Instead of relying on disparate systems and manual reporting, both buyer and supplier can access the same real-time data, enabling proactive problem-solving and informed decision-making. This transparency builds trust and strengthens the relationship. For instance, shared dashboards can show inventory levels, order fulfillment rates, and potential supply chain disruptions, allowing for timely interventions and collaborative mitigation strategies.
Key Performance Indicators for Supplier Performance Assessment
Effective supplier relationship management requires a robust system for tracking and evaluating supplier performance. BI tools are instrumental in this process, providing a centralized repository for relevant KPIs. These KPIs offer a clear and objective measure of a supplier’s effectiveness and reliability.
- On-Time Delivery Rate: This metric measures the percentage of orders delivered on or before the agreed-upon delivery date. A consistently high on-time delivery rate indicates a reliable supplier.
- Quality Rate: This KPI assesses the percentage of defect-free products or services received from a supplier. A high quality rate reflects a supplier’s commitment to quality control.
- Lead Time: The time it takes a supplier to fulfill an order, from receiving the order to delivering the goods. Shorter lead times indicate greater efficiency and responsiveness.
- Cost of Goods Sold (COGS): This reflects the direct costs associated with producing goods, providing insights into the supplier’s pricing and cost-effectiveness.
- Supplier Response Time: This measures the speed and efficiency with which a supplier responds to inquiries and resolves issues.
Identifying and Mitigating Supplier Risks with BI
Supply chain disruptions can have a significant impact on a business. BI helps proactively identify and mitigate potential supplier risks by analyzing various data points and predicting potential problems.BI can analyze historical data on supplier performance, market trends, and external factors like geopolitical events and natural disasters to identify potential vulnerabilities. For example, if a supplier consistently experiences delays during a specific season, BI can predict similar issues in future years, allowing the company to proactively seek alternative suppliers or adjust inventory levels.
Furthermore, BI can track key economic indicators relevant to the supplier’s region, such as inflation rates or currency fluctuations, to anticipate potential cost increases or disruptions. By analyzing this data, businesses can develop contingency plans to mitigate risks and ensure business continuity. Real-time monitoring of supplier performance allows for immediate identification of potential issues, enabling prompt corrective actions.
This proactive approach minimizes disruptions and maintains supply chain stability.
Risk Management and Mitigation: Using Business Intelligence To Improve Supply Chain Management Efficiency
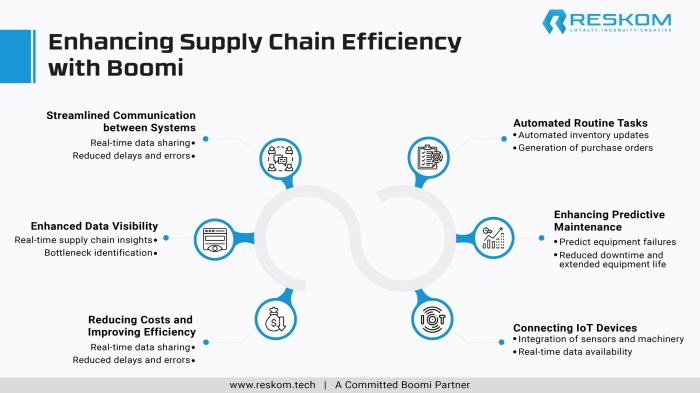
Business intelligence (BI) isn’t just about optimizing efficiency; it’s about proactively safeguarding your supply chain from potential disruptions. By leveraging data analysis and predictive modeling, businesses can identify vulnerabilities, anticipate risks, and implement mitigation strategies to ensure business continuity and resilience. This proactive approach, enabled by BI, transforms reactive firefighting into strategic risk management.BI identifies potential supply chain disruptions by analyzing vast datasets from various sources.
This includes historical sales data, supplier performance metrics, market trends, geopolitical events, and even weather patterns. By identifying patterns and anomalies, BI systems can flag potential bottlenecks, delays, or shortages before they impact operations. For example, a sudden spike in raw material prices, a drop in supplier reliability scores, or a predicted natural disaster in a key production area can all be detected and analyzed for their potential impact.
Identifying Potential Supply Chain Disruptions
BI tools utilize advanced analytics to identify potential disruptions. Real-time dashboards provide a clear overview of the supply chain’s health, highlighting areas of concern. Predictive modeling, using algorithms that learn from historical data, forecasts potential risks, such as supplier defaults, transportation delays, or demand surges. Anomaly detection algorithms flag unusual patterns that deviate from established norms, prompting further investigation.
For instance, a significant increase in lead times from a specific supplier might signal a potential problem requiring immediate attention. This proactive identification allows businesses to prepare and mitigate risks before they escalate into major crises.
Proactive Risk Management and Mitigation Strategies
Once potential disruptions are identified, BI facilitates the development and implementation of mitigation strategies. This could involve diversifying sourcing, building up safety stock of critical components, establishing alternative transportation routes, or negotiating contracts with more reliable suppliers. Scenario planning, a powerful BI capability, allows businesses to simulate various disruption scenarios and evaluate the effectiveness of different mitigation strategies.
For example, a business might simulate the impact of a port closure and compare the cost-effectiveness of rerouting shipments versus increasing inventory levels. The results guide decision-making and resource allocation.
Responding Effectively to Unexpected Events
Even with proactive risk management, unexpected events can occur. BI plays a crucial role in responding effectively to these unforeseen circumstances. Real-time data monitoring allows businesses to quickly assess the impact of the event on their supply chain. For instance, a sudden factory closure due to a natural disaster can be immediately identified, triggering pre-defined contingency plans.
BI dashboards provide a clear picture of the situation, enabling quick decision-making and resource allocation to minimize disruptions. Post-event analysis using BI helps to learn from the experience, refining risk mitigation strategies and improving future preparedness. This continuous improvement cycle is essential for building a truly resilient supply chain.
Measuring and Reporting Supply Chain Efficiency
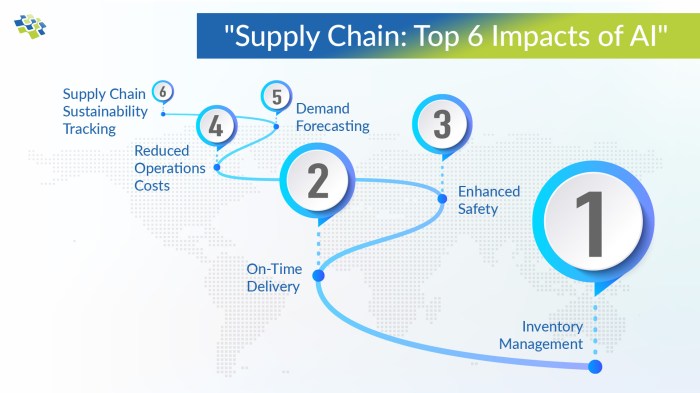
Understanding and tracking key performance indicators (KPIs) is crucial for optimizing your supply chain. Effective measurement allows businesses to identify bottlenecks, areas for improvement, and ultimately, boost profitability. Regular reporting provides a clear picture of performance, enabling data-driven decision-making and continuous enhancement.
Measuring supply chain efficiency involves analyzing various metrics, each offering a different perspective on overall performance. By combining these metrics, businesses gain a holistic view of their supply chain health and identify specific areas requiring attention.
Key Supply Chain Efficiency Metrics
Several key metrics provide a comprehensive assessment of supply chain efficiency. These metrics can be categorized into different areas such as inventory management, logistics, and customer service. Tracking these metrics allows for identification of strengths and weaknesses, facilitating strategic improvements.
Examples include:
- Inventory Turnover Ratio: This metric measures how efficiently inventory is managed. A higher ratio indicates efficient inventory management, while a lower ratio might suggest overstocking or slow-moving inventory. The formula is: Cost of Goods Sold / Average Inventory Value. For example, a company with a Cost of Goods Sold of $1 million and an average inventory value of $200,000 has an inventory turnover ratio of 5, indicating that it sells and replaces its inventory five times a year.
- On-Time Delivery Rate: This metric tracks the percentage of orders delivered on or before the promised delivery date. A high percentage demonstrates efficient logistics and reliable fulfillment processes. For example, if a company delivers 950 out of 1000 orders on time, its on-time delivery rate is 95%.
- Order Fulfillment Cycle Time: This measures the time taken from order placement to delivery. Shorter cycle times indicate streamlined processes and faster order fulfillment. This can be tracked from order placement to delivery confirmation, with benchmarks set based on industry standards and customer expectations. For instance, if an average order takes 3 days to fulfill, this can be compared against industry standards or customer expectations to identify potential improvements.
- Perfect Order Rate: This represents the percentage of orders fulfilled completely and accurately, without any errors. A high perfect order rate indicates a highly efficient and reliable supply chain. This encompasses aspects such as on-time delivery, correct order quantity, and accurate product condition. A company achieving a 98% perfect order rate is performing very well.
- Logistics Costs as a Percentage of Revenue: This metric shows the proportion of revenue spent on logistics activities. Lower percentages indicate efficient logistics management. If a company spends 10% of its revenue on logistics, it’s important to compare this figure to industry benchmarks to determine if it’s optimal.
Visual Representation of Supply Chain Metrics
A dashboard displaying key supply chain metrics provides a clear and concise overview of performance. Imagine a dashboard with several interactive charts and graphs.
One section could show a line graph illustrating the inventory turnover ratio over time, highlighting trends and potential anomalies. Another section might display a bar chart comparing the on-time delivery rate across different regions or product categories. A pie chart could visualize the proportion of revenue spent on various logistics activities. Key performance indicators (KPIs) like perfect order rate and order fulfillment cycle time could be displayed using gauges or numerical indicators with color-coding (green for exceeding targets, yellow for meeting targets, and red for falling short).
The dashboard should allow users to drill down into specific data points for further analysis and investigation.
Regular Reporting and Analysis for Continuous Improvement
Regular reporting and analysis are essential for continuous improvement. Weekly or monthly reports provide timely insights into supply chain performance, allowing for proactive adjustments. These reports should be analyzed to identify trends, bottlenecks, and areas requiring attention. The analysis should also incorporate benchmarking against industry standards and competitors to identify areas for improvement and best practices. This iterative process of measurement, analysis, and improvement drives ongoing optimization of the supply chain.
Case Studies
Real-world examples showcase the transformative power of Business Intelligence (BI) in optimizing supply chain management. By leveraging data-driven insights, companies across various sectors have significantly improved efficiency, reduced costs, and enhanced customer satisfaction. Let’s delve into specific case studies to understand the practical applications and impactful results.
Walmart’s Supply Chain Optimization
Walmart, a global retail giant, has extensively utilized BI to revolutionize its massive supply chain. Their system integrates data from various sources, including point-of-sale (POS) systems, supplier databases, and logistics tracking data. This comprehensive data integration allows for real-time visibility into inventory levels, demand patterns, and logistical bottlenecks. Walmart’s BI system enables predictive analytics, forecasting future demand with impressive accuracy.
This allows for optimized inventory management, minimizing stockouts and reducing waste from excess inventory. Furthermore, the data-driven insights improve logistics and transportation planning, resulting in more efficient delivery routes and reduced transportation costs. The outcome? Improved on-shelf availability, reduced waste, and significant cost savings across the entire supply chain. The success of Walmart’s BI implementation demonstrates the scalability and impact of data-driven decision-making in a large, complex supply chain.
DHL’s Enhanced Logistics and Transportation
DHL, a leading global logistics company, employs BI to optimize its vast network of transportation and delivery services. Their BI solutions analyze real-time data from various sources, including vehicle tracking systems, weather forecasts, and traffic conditions. This data is used to create dynamic routing algorithms that optimize delivery routes, considering factors like traffic congestion and weather disruptions. The BI system also helps in predicting potential delays and proactively mitigating disruptions.
By leveraging predictive analytics, DHL can optimize resource allocation, ensuring timely deliveries and enhancing customer satisfaction. The company also uses BI to analyze delivery performance metrics, identifying areas for improvement and streamlining its operations. This leads to cost reductions through improved fuel efficiency, reduced transportation time, and optimized workforce deployment. DHL’s successful integration of BI demonstrates how real-time data analysis and predictive modeling can significantly enhance logistics efficiency and customer service in a highly dynamic environment.