The role of artificial intelligence in modern business intelligence systems is rapidly transforming how businesses operate. No longer a futuristic concept, AI is actively enhancing data analysis, prediction, and decision-making, leading to unprecedented levels of efficiency and insight. From automating tedious tasks to uncovering hidden patterns in massive datasets, AI is proving to be a game-changer, pushing the boundaries of what’s possible in the world of business intelligence.
This evolution isn’t just about faster processing; it’s about unlocking a deeper understanding of customer behavior, market trends, and operational efficiencies. This article dives into the multifaceted ways AI is reshaping business intelligence, exploring its impact on data processing, visualization, predictive modeling, and ultimately, strategic decision-making. Prepare to be amazed by the potential of AI to revolutionize your business.
AI-Driven Data Processing and Analysis in Modern Business Intelligence
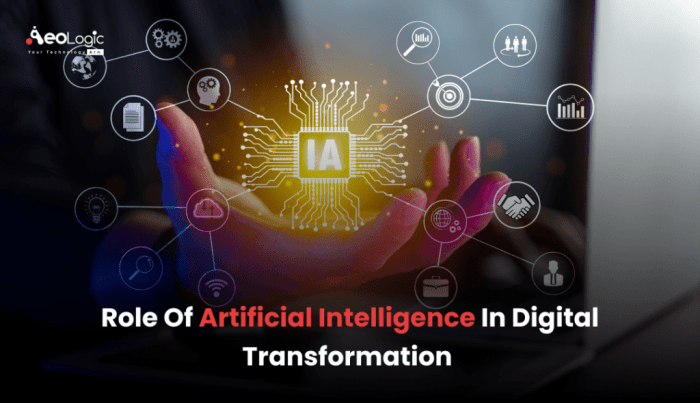
The integration of artificial intelligence (AI) is revolutionizing modern business intelligence (BI) systems, transforming how businesses collect, process, and interpret data to gain actionable insights. AI algorithms are no longer a futuristic concept; they are actively streamlining data workflows, enhancing analytical capabilities, and ultimately driving more effective decision-making. This enhanced efficiency translates to faster turnaround times, more accurate predictions, and a deeper understanding of complex business trends.AI algorithms significantly improve data cleaning, transformation, and preparation processes within BI systems.
AI’s impact on modern business intelligence is undeniable, offering predictive analytics and automated insights that streamline operations. This is especially crucial for businesses leveraging integrated systems like those found in erp ecommerce , where AI can optimize inventory management, personalize customer experiences, and forecast sales trends with unprecedented accuracy. Ultimately, AI empowers better decision-making across the entire business intelligence ecosystem.
Traditional methods often rely on manual processes, prone to errors and requiring significant time investment. AI, however, automates many of these tasks. Machine learning models can identify and correct inconsistencies, handle missing values, and transform data into formats suitable for analysis far more efficiently and accurately than manual processes. For instance, AI can automatically detect and correct typos in customer addresses or identify and flag outliers in sales data, ensuring data quality before analysis even begins.
AI Models for Predictive Analytics in Business Intelligence
Various AI models power predictive analytics within BI systems, each offering unique strengths depending on the specific business problem. These models leverage historical data to forecast future trends and outcomes, providing businesses with a significant competitive advantage.
- Regression Models: These models predict a continuous outcome variable (e.g., sales revenue) based on one or more predictor variables (e.g., marketing spend, seasonality). A linear regression model, for example, could predict future sales based on past sales figures and advertising expenditure. This allows businesses to optimize their marketing strategies for maximum ROI.
- Classification Models: These models predict a categorical outcome (e.g., customer churn, fraud detection). A logistic regression model could predict the likelihood of a customer churning based on their usage patterns and demographics. This enables proactive customer retention strategies.
- Time Series Models: These models analyze data points collected over time to identify trends and patterns, forecasting future values. An ARIMA model, for example, could be used to predict future demand for a product based on historical sales data. This helps optimize inventory management and production planning.
- Neural Networks: These complex models can analyze vast datasets to identify intricate patterns and relationships that might be missed by simpler models. Deep learning neural networks, for example, are used in image recognition for quality control in manufacturing or in natural language processing for sentiment analysis of customer feedback.
Comparison of Traditional BI and AI-Powered Approaches
Traditional BI methods often involve manual data manipulation, static reporting, and limited predictive capabilities. AI-powered BI, on the other hand, offers significant improvements in speed and accuracy. AI algorithms can process massive datasets far quicker than humans, identifying patterns and insights that would be impossible to detect manually. Furthermore, AI’s predictive capabilities allow for more accurate forecasting and proactive decision-making, leading to better business outcomes.
For example, analyzing sales data from the last five years using traditional methods might take weeks, while an AI-powered system could complete the same analysis in hours, providing more timely and accurate predictions.
AI Acceleration of Insight Identification
Imagine a large e-commerce company with millions of customer transactions daily. Traditional BI methods would struggle to identify meaningful patterns and insights from this data volume. However, an AI-powered system could quickly analyze this data, identifying, for example, specific product combinations frequently purchased together, predicting future demand for these products, and optimizing inventory management accordingly. This allows the company to proactively adjust its marketing strategies, improve supply chain efficiency, and ultimately boost sales and profitability.
The AI system might also identify emerging customer segments based on purchasing behavior and demographics, allowing for targeted marketing campaigns and personalized recommendations, leading to increased customer engagement and loyalty.
AI’s Role in Enhancing Data Visualization and Reporting
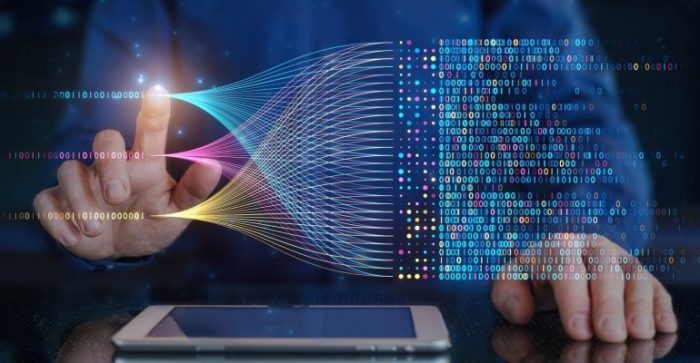
The integration of artificial intelligence (AI) is revolutionizing business intelligence (BI) systems, moving beyond simple data analysis to create more insightful, personalized, and interactive experiences. AI is no longer just a tool for processing data; it’s becoming the engine driving the creation and interpretation of compelling visualizations and reports. This allows businesses to make faster, more informed decisions based on a deeper understanding of their data.AI significantly enhances data visualization and reporting by automating tasks, personalizing insights, and uncovering hidden patterns previously invisible to human analysts.
This leads to improved decision-making, increased efficiency, and a competitive edge in today’s data-driven world.
Personalized Dashboards and Reports
AI algorithms can analyze user behavior and preferences to tailor dashboards and reports to individual needs. For example, a sales manager might see a dashboard highlighting key performance indicators (KPIs) specific to their region and product line, while a marketing executive might see a dashboard focused on campaign performance and customer segmentation. This personalization ensures that users only see the information most relevant to their roles and responsibilities, increasing efficiency and reducing information overload.
AI can achieve this personalization by analyzing past report interactions, user roles, and even real-time data to dynamically adjust the displayed information.
AI-Powered Visualization Tools
Several AI-powered tools enhance the understanding of complex data patterns. For instance, tools utilizing machine learning can automatically identify clusters in data, revealing previously unknown customer segments or product groupings. These tools often employ techniques like dimensionality reduction (e.g., t-SNE) to visualize high-dimensional data in a comprehensible two- or three-dimensional space. Another example is the use of AI to create interactive maps that visually represent geographical data, highlighting trends and outliers.
These interactive maps can dynamically adjust their focus based on user selection, providing granular details on demand.
AI-Driven Narrative Generation
AI can go beyond simply creating visualizations; it can also generate insightful narratives from the data. Natural Language Generation (NLG) algorithms can analyze data visualizations and automatically produce written summaries or reports, highlighting key findings and trends. Imagine an AI summarizing sales performance, stating, “Sales in the Northeast region exceeded expectations by 15% this quarter, primarily driven by the success of product X.” This automated reporting saves analysts significant time and effort, allowing them to focus on higher-level strategic tasks.
Interactive and Dynamic Reports
AI enables the creation of interactive and dynamic reports that respond to user queries in real-time. Instead of static reports, users can interact with visualizations, drilling down into specific data points or exploring different perspectives. For example, a user might click on a specific bar in a chart to see detailed information about the underlying data points, or they might use natural language to ask questions about the data, such as “What were the top three performing products last month?” The AI-powered report would then dynamically update to provide the requested information.
Comparison of AI-Powered Data Visualization Techniques, The role of artificial intelligence in modern business intelligence systems
Technique | Strengths | Weaknesses | Example Application |
---|---|---|---|
Clustering Algorithms (e.g., k-means) | Identifies hidden patterns and groups in data; useful for customer segmentation. | Requires pre-defining the number of clusters; can be sensitive to outliers. | Grouping customers based on purchasing behavior to personalize marketing campaigns. |
Dimensionality Reduction (e.g., t-SNE) | Visualizes high-dimensional data in lower dimensions, making complex datasets easier to understand. | Can be computationally expensive; the resulting visualization might not always be easily interpretable. | Visualizing gene expression data to identify relationships between genes. |
Natural Language Generation (NLG) | Automates report writing, saving time and effort; provides concise summaries of findings. | Can struggle with complex or nuanced data; requires careful calibration to ensure accuracy. | Generating automated sales reports highlighting key trends and performance indicators. |
Interactive Dashboards | Allows users to explore data dynamically; provides a more engaging and insightful experience. | Can be complex to design and implement; requires careful consideration of user interface design. | Creating a dashboard for real-time monitoring of website traffic and user engagement. |
AI-Powered Predictive Modeling and Forecasting in Business Intelligence: The Role Of Artificial Intelligence In Modern Business Intelligence Systems
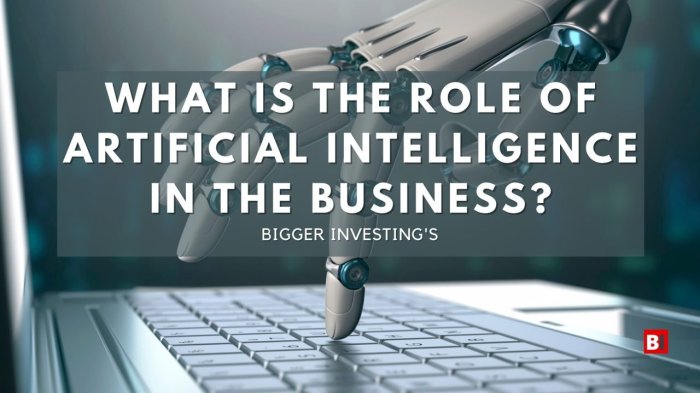
Predictive modeling, powered by artificial intelligence, is revolutionizing business intelligence, enabling organizations to move beyond descriptive analytics and delve into the realm of proactive decision-making. By leveraging historical data and advanced algorithms, businesses can anticipate future trends, optimize operations, and gain a significant competitive edge. However, the journey to accurate and ethical AI-driven forecasting is fraught with challenges that need careful consideration.
Challenges in Building Accurate Predictive Models
Building accurate predictive models using AI in business intelligence presents several key hurdles. Data quality is paramount; incomplete, inconsistent, or biased data can lead to inaccurate predictions. The complexity of real-world business scenarios often defies simple modeling; factors like seasonality, external market forces, and unforeseen events can significantly impact accuracy. Furthermore, choosing the appropriate AI algorithm for a specific task requires careful consideration of the data characteristics and the desired outcome.
Finally, model interpretability remains a challenge; understandingwhy* a model makes a specific prediction is crucial for trust and effective decision-making, yet many advanced algorithms, like deep learning models, are notoriously opaque. For example, a model predicting customer churn might fail if it doesn’t account for changes in customer service policies or competitor actions.
Ethical Considerations in AI-Driven Predictive Modeling
The ethical implications of using AI for predictive modeling are substantial. Bias in the training data can lead to discriminatory outcomes, perpetuating existing inequalities. For instance, a loan application prediction model trained on historical data reflecting existing biases might unfairly deny loans to certain demographic groups. Privacy concerns are paramount; using personal data for predictive modeling requires careful consideration of data protection regulations and ethical guidelines.
Transparency and explainability are crucial for building trust and ensuring accountability. Organizations must be prepared to explain how their AI models work and address potential concerns regarding fairness and bias. Furthermore, the potential for misuse of predictive models, such as manipulating results for personal gain, must be addressed proactively.
Deploying and Monitoring AI-Powered Forecasting Models
Deploying and monitoring AI-powered forecasting models involves a structured process. Initially, the model is integrated into the existing business intelligence system, often through APIs or custom integrations. This requires careful consideration of data flow, security, and scalability. Continuous monitoring is essential to track model performance and identify potential issues. Regular model retraining is necessary to account for changes in data patterns and external factors.
Performance metrics, such as accuracy, precision, and recall, should be closely monitored. Anomaly detection mechanisms can alert users to significant deviations from expected performance. For example, a sales forecasting model might require retraining after a major marketing campaign or a change in economic conditions. A robust feedback loop, incorporating human expertise and real-world observations, is crucial for continuous improvement.
Comparison of AI Algorithms for Forecasting
Various AI algorithms can be employed for forecasting sales, customer churn, or market trends. Linear regression is a simple, interpretable method suitable for linear relationships. Time series analysis methods, such as ARIMA, are well-suited for forecasting time-dependent data. Machine learning algorithms like Random Forests and Gradient Boosting Machines offer high accuracy but can be less interpretable. Neural networks, including Recurrent Neural Networks (RNNs) and Long Short-Term Memory (LSTM) networks, excel at capturing complex patterns in sequential data, but require substantial computational resources and expertise.
The choice of algorithm depends on the specific forecasting task, data characteristics, and the desired level of interpretability. For instance, predicting short-term sales fluctuations might benefit from simpler models like ARIMA, while long-term market trend prediction could leverage the power of deep learning.
Implementing an AI-Driven Predictive Maintenance System
Implementing an AI-driven predictive maintenance system in a manufacturing company follows a structured approach.
- Data Acquisition: Collect sensor data from machines, including vibration, temperature, and pressure readings.
- Data Preprocessing: Clean and prepare the data, handling missing values and outliers. Feature engineering might involve creating new variables from existing ones (e.g., calculating vibration frequency).
- Model Selection and Training: Choose an appropriate machine learning algorithm, such as Random Forest or Support Vector Machine (SVM), and train it on the prepared data to predict machine failures.
- Model Deployment: Integrate the trained model into the manufacturing system, allowing for real-time predictions.
- Monitoring and Maintenance: Continuously monitor the model’s performance and retrain it periodically with new data. This ensures the model adapts to changing conditions and maintains accuracy.
For example, a manufacturer could use sensor data from a production line to predict when a specific machine is likely to fail, allowing for proactive maintenance and minimizing downtime. This prevents unexpected breakdowns and improves overall operational efficiency.
The Impact of AI on Business Decision-Making Processes
AI is rapidly transforming how businesses make decisions, moving beyond gut feelings and intuition to data-driven insights. This shift empowers organizations to make faster, more informed choices, ultimately leading to improved efficiency and profitability. The integration of AI into business intelligence systems allows for the automation of complex processes, the identification of previously unseen patterns, and the prediction of future trends – all crucial elements in effective decision-making.AI assists in automating decision-making processes by handling vast amounts of data that would be impossible for humans to process manually.
Through machine learning algorithms, AI can identify patterns and correlations, analyze risks and opportunities, and even recommend specific actions. This automation frees up human resources to focus on higher-level strategic tasks, requiring creativity and critical thinking, rather than being bogged down in data analysis. For example, AI can automate routine tasks like invoice processing, inventory management, and customer service responses, allowing human employees to focus on more complex decision-making processes.
AI Support for Strategic Decision-Making
AI’s ability to analyze massive datasets allows it to uncover hidden opportunities and risks that might otherwise go unnoticed. For instance, by analyzing customer purchase history, social media sentiment, and market trends, AI can predict future demand for products or services, enabling businesses to optimize inventory, pricing, and marketing strategies. Similarly, AI can identify potential risks, such as supply chain disruptions or changes in consumer preferences, allowing businesses to proactively mitigate these threats.
Consider a retail company using AI to analyze sales data and predict a surge in demand for a particular product during a specific holiday season. This allows them to adjust their supply chain, ensuring sufficient inventory to meet the demand and avoid stockouts, maximizing profits and customer satisfaction. Conversely, AI could identify a decline in customer engagement with a particular product line, prompting the company to investigate the underlying reasons and potentially adjust its marketing strategy or product development roadmap.
Integrating AI-Driven Insights into Existing Frameworks
Successfully integrating AI-driven insights requires a structured approach. It’s not simply a matter of plugging in an AI tool; it requires a thoughtful consideration of how AI outputs can be incorporated into existing decision-making processes. This involves establishing clear goals for AI implementation, selecting appropriate AI tools and techniques, and ensuring the integration aligns with the company’s overall strategy.
Furthermore, it’s crucial to establish processes for validating AI-generated insights and incorporating them into the existing decision-making framework. This often requires collaboration between data scientists, business analysts, and decision-makers to ensure that the AI insights are properly interpreted and acted upon. For example, a company might integrate AI-driven market forecasts into its annual budgeting process, using the AI’s predictions to inform resource allocation and investment decisions.
AI’s Impact Across Industries
AI’s influence on decision-making spans various industries. In finance, AI-powered algorithms assess credit risk, detect fraud, and optimize investment portfolios. In healthcare, AI aids in diagnosis, treatment planning, and drug discovery. In manufacturing, AI optimizes production processes, predicts equipment failures, and improves supply chain management. The impact is consistent across these sectors: increased efficiency, reduced costs, and improved decision-making accuracy.
For example, in the logistics industry, AI optimizes delivery routes, predicts delivery times, and manages warehouse operations, leading to significant cost savings and improved customer satisfaction.
Benefits and Drawbacks of AI in Business Decision-Making
Before implementing AI, it’s essential to understand both its potential benefits and limitations.
The potential benefits are substantial:
- Improved accuracy and speed of decision-making
- Enhanced risk management and opportunity identification
- Increased efficiency and productivity
- Better resource allocation and cost optimization
- Improved customer experience
However, there are also drawbacks to consider:
- High initial investment costs
- Need for specialized expertise and skills
- Potential for bias in algorithms
- Data privacy and security concerns
- Lack of transparency and explainability in some AI models
AI and the Future of Business Intelligence Systems
The integration of artificial intelligence (AI) is rapidly transforming business intelligence (BI) systems, paving the way for a future where data-driven decision-making is faster, more accurate, and more insightful than ever before. This evolution isn’t just about incremental improvements; it’s a fundamental shift in how businesses understand and utilize their data. The coming years will witness a convergence of advanced AI techniques with sophisticated BI platforms, leading to unprecedented opportunities and challenges.
Emerging trends in AI, such as explainable AI (XAI), generative AI, and advancements in natural language processing (NLP), are poised to revolutionize future BI systems. These advancements promise to address some of the limitations of current systems, making them more accessible, understandable, and ultimately, more valuable to businesses of all sizes.
Emerging AI Trends and Their Impact on Future BI Systems
The landscape of AI is constantly evolving, with new techniques and applications emerging at a rapid pace. Explainable AI (XAI), for example, focuses on making the decision-making processes of AI models transparent and understandable to humans. This is crucial in BI, where trust and confidence in the insights generated are paramount. Generative AI, capable of creating new data instances that resemble real-world data, can be used to fill in data gaps, improve data quality, and even simulate different scenarios for better forecasting.
Finally, advancements in NLP are enabling more natural and intuitive interactions with BI systems, making them accessible to a wider range of users. Consider a scenario where a business user can simply ask a question in natural language, such as “What were our sales in Q3 compared to last year?”, and receive a clear, concise, and visually appealing answer without needing to navigate complex dashboards or write SQL queries.
This improved accessibility and ease of use will significantly increase the adoption and utilization of BI tools across organizations.
Challenges and Opportunities in AI Adoption for Business Intelligence
Widespread adoption of AI in BI presents both exciting opportunities and significant challenges. One key challenge is the need for high-quality, clean data. AI models are only as good as the data they are trained on, and inaccurate or incomplete data can lead to flawed insights. Another challenge lies in the potential for bias in AI algorithms.
If the data used to train an AI model contains biases, those biases will likely be reflected in the model’s outputs. Addressing these challenges requires careful data management, rigorous model validation, and a strong focus on ethical considerations. However, the opportunities are equally compelling. AI can automate many time-consuming tasks, such as data cleaning, preprocessing, and report generation, freeing up human analysts to focus on more strategic activities.
AI can also uncover hidden patterns and insights in data that would be impossible for humans to detect manually, leading to more effective decision-making. The successful integration of AI into BI systems will ultimately depend on a careful balance between leveraging the technology’s potential and mitigating its risks.
The Role of Human Expertise in AI-Powered Business Intelligence
Despite the rapid advancements in AI, the role of human expertise remains crucial in AI-powered BI systems. Humans are needed to guide the development and implementation of AI models, ensuring that they are aligned with business goals and ethical considerations. Human analysts are also essential for interpreting the insights generated by AI models, adding context, and making strategic recommendations based on the data.
The future of BI is not about replacing humans with AI, but rather about empowering humans with AI to make better decisions. Humans bring critical thinking, creativity, and domain expertise that are currently unmatched by AI. The collaborative relationship between humans and AI will be the key to unlocking the full potential of AI-powered BI.
A Conceptual Framework for a Next-Generation BI System
A next-generation BI system leveraging the full potential of AI would incorporate several key features. First, it would feature a robust data integration and management layer capable of handling large volumes of diverse data sources. Second, it would utilize advanced AI algorithms for data cleaning, preprocessing, and analysis, including techniques like machine learning and deep learning. Third, it would provide intuitive and user-friendly interfaces, leveraging NLP for natural language querying and interaction.
Fourth, it would incorporate XAI techniques to ensure transparency and explainability of AI-driven insights. Fifth, it would integrate advanced visualization tools to present complex data in a clear and compelling manner. Such a system would be adaptable and scalable, allowing businesses to easily incorporate new data sources and AI techniques as they become available. Imagine a system that can automatically detect anomalies in sales data, provide explanations for those anomalies, and suggest potential actions to mitigate any negative impacts – all without requiring extensive manual intervention.
The Impact of Natural Language Processing on Business Interaction with BI Systems
Advancements in NLP are transforming how businesses interact with their BI systems. Instead of relying on complex queries or navigating cumbersome dashboards, users can simply ask questions in natural language. For instance, a sales manager could ask, “What are the top three products driving revenue growth in the Midwest region this quarter, and what are the contributing factors?”, and receive a detailed response with relevant charts and graphs.
This intuitive interaction significantly improves user experience, making BI accessible to a wider range of users, regardless of their technical expertise. The efficiency gains are also substantial, as users can obtain the information they need quickly and easily, without needing to spend time learning complex software or writing queries. This empowers users to make data-driven decisions more rapidly and effectively, ultimately leading to improved business outcomes.
The scenario illustrates a future where the barrier between business users and data insights is significantly lowered, fostering a more data-literate and efficient organization.