Strategic data insights are the key to unlocking hidden business potential. This isn’t just about crunching numbers; it’s about transforming raw data into actionable intelligence that drives strategic decision-making. We’ll explore how to identify key performance indicators (KPIs), ethically collect and prepare data, and utilize powerful methods to extract meaningful insights. From data mining techniques to predictive modeling, we’ll uncover how to communicate these insights effectively, ultimately measuring their impact on achieving significant business outcomes.
This deep dive into strategic data insights will equip you with the knowledge and tools to leverage data for competitive advantage. We’ll cover everything from defining what strategic data insights actually are to implementing them and measuring their success, making this a comprehensive guide for anyone looking to harness the power of data-driven decision-making.
Defining Strategic Data Insights
Strategic data insights are the actionable intelligence derived from analyzing data, providing a clear competitive advantage and informing crucial business decisions. Unlike simple data analysis that might reveal trends, strategic data insights offer a deeper understanding ofwhy* those trends exist, predicting future outcomes and suggesting effective interventions. They are the key to unlocking hidden potential and driving impactful change within an organization.Strategic data insights differ from other forms of data analysis in their focus and application.
While descriptive analytics simply summarize past data, and predictive analytics forecast future trends, strategic data insights go further, uncovering the underlying causal relationships and providing prescriptive guidance. They are not just about understanding what happened or what might happen, but about understanding
- why* it happened and
- how* to influence future outcomes. This requires a more sophisticated analytical approach, often involving advanced techniques like machine learning and statistical modeling.
Sources of Strategic Data Insights
Strategic data insights can be gleaned from a variety of sources, both internal and external to an organization. Effectively leveraging these diverse data streams is crucial for gaining a holistic and comprehensive understanding of the business environment.
- Internal Data: This includes operational data from CRM systems, sales figures, marketing campaign performance metrics, customer service interactions, and internal communications. Analyzing this data can reveal patterns in customer behavior, identify areas for process improvement, and optimize resource allocation.
- External Data: This encompasses market research reports, competitor analysis, economic indicators, social media sentiment, and news articles. Analyzing external data provides valuable context and allows organizations to anticipate market shifts, understand competitive landscapes, and adapt their strategies accordingly. For example, analyzing social media sentiment around a new product launch can provide valuable early feedback and inform marketing adjustments.
- Third-Party Data: This includes data purchased from specialized data providers, offering demographic information, consumer behavior patterns, and market trends. This type of data can supplement internal and external data, enriching the analysis and providing a more complete picture. For instance, a retailer might use third-party data to identify new customer segments and target marketing campaigns more effectively.
Identifying Key Performance Indicators (KPIs) for Strategic Insights
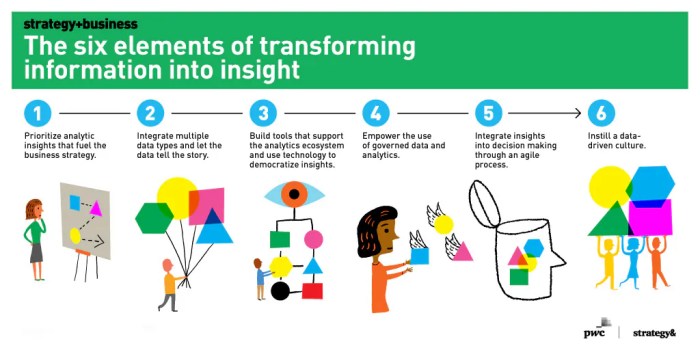
Understanding and tracking the right Key Performance Indicators (KPIs) is crucial for any e-commerce business aiming for sustainable growth. KPIs provide a quantifiable measure of progress towards strategic goals, allowing businesses to make data-driven decisions and optimize their operations. By focusing on the most impactful metrics, companies can efficiently allocate resources and enhance overall performance.
Five Crucial KPIs for an E-commerce Business, Strategic data insights
Choosing the right KPIs depends on the specific business objectives. However, several metrics consistently prove vital for e-commerce success. The following table Artikels five crucial KPIs, their associated metrics, data sources, and strategic goals.
KPI | Metric | Data Source | Strategic Goal |
---|---|---|---|
Conversion Rate | Percentage of website visitors who complete a desired action (e.g., purchase) | Website analytics (Google Analytics, etc.) | Increase sales and revenue |
Average Order Value (AOV) | Total revenue divided by the number of orders | E-commerce platform data | Boost profitability by encouraging higher-value purchases |
Customer Acquisition Cost (CAC) | Total marketing and sales expenses divided by the number of new customers acquired | Marketing and sales data, CRM | Optimize marketing spend and improve ROI |
Customer Lifetime Value (CLTV) | Predicted revenue generated by a single customer over their entire relationship with the business | Sales data, customer behavior data | Identify and retain high-value customers |
Website Traffic | Number of unique visitors and page views | Website analytics | Expand brand reach and increase sales opportunities |
Dashboard Designs for Visualizing KPIs
Effective data visualization is key to understanding and acting upon KPI data. Different dashboards cater to specific audiences and needs.
Dashboard 1: Executive Summary Dashboard
Target Audience: C-suite executives, board members. Layout: A concise overview featuring only the most critical KPIs (Conversion Rate, AOV, and Revenue). Key Visual Elements: Large, clear charts (e.g., line charts showing trends over time, bar charts comparing performance across different periods) with minimal text. A focus on high-level trends and overall performance.
Dashboard 2: Marketing Performance Dashboard
Target Audience: Marketing team. Layout: Detailed view of marketing campaign performance. Key Visual Elements: Charts showing CAC, website traffic sources, and conversion rates broken down by marketing channel. Use of geographic maps to visualize regional performance. Interactive elements to drill down into specific campaigns.
Dashboard 3: Customer Insights Dashboard
Target Audience: Customer service and sales teams. Layout: Focus on customer behavior and retention. Key Visual Elements: Charts visualizing CLTV, customer segmentation, and repeat purchase rates. Use of heatmaps to identify high-value customers and potential churn risks. Interactive elements to access individual customer profiles.
Leading, Lagging, and Process KPIs
Understanding the different types of KPIs is crucial for effective strategic decision-making. Leading indicators predict future performance, lagging indicators reflect past performance, and process KPIs measure the efficiency of operational processes.
Leading KPIs (e.g., website traffic, marketing campaign engagement) provide early warning signs of potential issues or opportunities. For example, a sudden drop in website traffic might signal a problem with or marketing campaigns, allowing for timely intervention.
Lagging KPIs (e.g., revenue, customer churn rate) reflect the results of past actions. Analyzing lagging KPIs helps understand the overall impact of strategies implemented previously. For instance, a high customer churn rate might indicate issues with customer service or product quality.
Process KPIs (e.g., order fulfillment time, customer service response time) measure the efficiency of internal processes. Improving process KPIs can directly impact leading and lagging KPIs. For example, faster order fulfillment can lead to higher customer satisfaction and repeat purchases. By monitoring all three types, businesses gain a holistic view of their performance and identify areas for improvement.
Unlocking strategic data insights requires the right tools and infrastructure. This Black Friday, supercharge your data analysis capabilities with incredible savings on managed WordPress hosting by checking out the amazing deals on wpengine black friday offers. Investing in robust hosting is crucial for efficiently processing and analyzing large datasets, ultimately leading to sharper, more effective strategic data insights.
Data Collection and Preparation for Insight Generation
Unlocking the power of strategic data insights hinges on a robust and ethical data foundation. Gathering, cleaning, and preparing data is not merely a technical exercise; it’s a crucial step that directly impacts the reliability and validity of your insights, ultimately influencing strategic decision-making. This process demands meticulous attention to detail and a strong ethical compass.Data collection and preparation for insightful analysis involves several critical steps, each with its own set of challenges and best practices.
Ignoring these steps can lead to inaccurate conclusions and flawed strategies. This section Artikels the key considerations for ensuring data quality and generating reliable insights.
Ethical Considerations in Data Collection and Utilization
Ethical data handling is paramount. Collecting and using data without informed consent or violating privacy regulations can have severe legal and reputational consequences. Transparency with data subjects about how their data will be used is essential. Anonymization and data security measures should be implemented to protect sensitive information. Organizations should adhere to relevant data privacy regulations like GDPR and CCPA, ensuring compliance throughout the entire data lifecycle.
For example, a company collecting customer purchase history must clearly state this in its privacy policy and obtain consent before using this data for targeted advertising. Failure to do so can lead to hefty fines and damage to brand trust.
Best Practices for Ensuring Data Quality and Accuracy
Data quality directly impacts the reliability of insights. Best practices include establishing clear data definitions and validation rules upfront. Regular data audits and quality checks are crucial to identify and correct inconsistencies or errors. Data governance frameworks should be implemented to ensure data accuracy, consistency, and completeness. For instance, establishing standardized data entry protocols can minimize human error, while automated data validation checks can catch inconsistencies in real-time.
A robust data governance framework ensures everyone understands data definitions, access protocols, and quality control procedures.
Step-by-Step Procedure for Data Cleaning and Preparation
Cleaning and preparing large datasets for analysis is a multi-step process. It often involves handling missing values, outliers, and inconsistencies.
- Data Inspection and Profiling: Begin by thoroughly inspecting the dataset for its structure, data types, and identifying potential issues like missing values, inconsistencies, and outliers. This step involves using descriptive statistics and data visualization techniques to understand the data’s characteristics.
- Handling Missing Values: Missing data can significantly impact analysis. Strategies include deletion (if the missing data is minimal and random), imputation (replacing missing values with estimated values based on other data points – using methods like mean, median, or more sophisticated techniques like k-Nearest Neighbors), or using specialized analytical methods designed to handle missing data.
- Outlier Detection and Treatment: Outliers are data points that significantly deviate from the rest of the data. Detection methods include box plots, scatter plots, and statistical measures like Z-scores. Treatment options include removal (if outliers are due to errors), transformation (e.g., logarithmic transformation to reduce the impact of extreme values), or using robust statistical methods less sensitive to outliers.
- Data Transformation and Cleaning: This involves converting data into a suitable format for analysis. This may include data type conversions, standardizing units, and correcting inconsistencies in data entries. For example, converting dates to a consistent format or ensuring that all currency values are in the same unit.
- Data Validation and Verification: After cleaning, validate the data for accuracy and consistency. This involves comparing cleaned data against original data sources and verifying that the transformations and cleaning steps have not introduced new errors.
For example, consider a dataset of customer sales data with missing values for certain transactions and outliers representing unusually high purchase amounts. By employing imputation techniques to fill missing values and using robust statistical methods to account for outliers, we can generate more accurate insights into customer purchasing behavior. Failing to address these issues would lead to skewed results and unreliable conclusions.
Methods for Extracting Strategic Insights: Strategic Data Insights
Unlocking the true potential of your data requires more than just collecting it; you need to extract meaningful insights that drive strategic decisions. This involves employing sophisticated analytical techniques and visualizing the results in a way that’s both clear and compelling. Let’s explore the key methods that turn raw data into actionable intelligence.
Data Mining Techniques for Uncovering Hidden Patterns
Data mining, a powerful subset of data science, utilizes various algorithms to sift through massive datasets, identifying hidden patterns, trends, and anomalies that might otherwise go unnoticed. These patterns can reveal valuable information about customer behavior, market trends, operational inefficiencies, and more. For instance, association rule mining can uncover unexpected relationships between products purchased together, allowing businesses to optimize product placement and cross-selling strategies.
Clustering algorithms can group similar customers based on demographics and purchasing habits, enabling targeted marketing campaigns. Anomaly detection can flag unusual transactions, potentially indicating fraud or other security breaches. By applying these techniques, businesses can gain a competitive edge by making data-driven decisions based on insights that would be impossible to discover through manual analysis.
Data Visualization Methods for Communicating Strategic Insights
Effective communication of strategic insights is crucial for driving action. Different visualization methods excel at conveying different types of information. Bar charts are ideal for comparing discrete categories, such as sales performance across different regions. Scatter plots effectively illustrate the relationship between two continuous variables, for example, showing the correlation between advertising spend and sales revenue. Heatmaps are excellent for visualizing large datasets with multiple variables, such as customer satisfaction ratings across different product features.
While bar charts offer simplicity and clarity, scatter plots reveal correlations, and heatmaps provide a comprehensive overview of complex relationships. The choice of visualization method should always align with the specific insight being communicated and the target audience. For example, a simple bar chart might be sufficient for a high-level executive summary, while a more complex heatmap might be necessary for a detailed technical report.
Predictive Modeling for Forecasting Future Trends
Predictive modeling leverages historical data and statistical techniques to forecast future outcomes. This allows businesses to proactively adapt to changing market conditions and make informed strategic decisions. For example, a retail company might use predictive modeling to forecast demand for specific products based on past sales data, seasonality, and economic indicators. This allows them to optimize inventory levels, avoid stockouts, and minimize waste.
Similarly, a financial institution might use predictive modeling to assess credit risk and identify potential defaults. These models can incorporate a wide range of variables, including demographic information, credit history, and economic conditions, to generate accurate predictions. The accuracy of predictive models depends on the quality and quantity of the data used, as well as the sophistication of the modeling techniques employed.
Regular model evaluation and refinement are crucial to maintain accuracy and relevance over time. For example, a model predicting online shopping behavior might need to be updated frequently to account for changes in consumer preferences and technological advancements.
Communicating and Implementing Strategic Insights

Transforming raw data into actionable strategies is only half the battle. Effectively communicating those insights to stakeholders and then successfully implementing them is crucial for achieving tangible business results. This requires a blend of clear communication, compelling storytelling, and a well-defined implementation plan.Presenting complex data insights to a non-technical audience demands a strategic approach. Simply throwing numbers and charts at them won’t suffice; instead, focus on translating complex information into easily digestible narratives.
Designing Presentations for Non-Technical Audiences
A successful presentation should prioritize clarity and simplicity. Use visuals like charts and graphs that are easy to understand, avoiding cluttered designs or overly technical jargon. Instead of focusing on intricate details, highlight the key takeaways and their implications for the business. For instance, instead of showing a complex regression analysis, summarize the key finding – “Increased marketing spend in Q3 led to a 15% rise in customer acquisition.” Use concise bullet points to reinforce key messages and keep the language clear and accessible.
Think of it as translating data into a language everyone can understand. A strong visual representation of the data, such as a simple bar chart comparing performance before and after a specific initiative, is far more impactful than a dense spreadsheet.
The Power of Storytelling in Data Presentation
Data, on its own, can be dry and unengaging. However, framing data within a compelling narrative transforms it into a powerful tool. By weaving data points into a story that resonates with the audience’s understanding and aspirations, you create a more memorable and impactful presentation. Consider the classic narrative arc: introduce the challenge, highlight the data-driven solution, and showcase the positive outcome.
For example, instead of stating “Customer churn decreased by 10%,” you could say, “By implementing a new customer feedback system, we identified the root causes of customer dissatisfaction and reduced churn by 10%, resulting in significant cost savings and improved customer loyalty.” This approach humanizes the data and makes it more relatable.
Successful Implementations of Strategic Insights
Successful implementation hinges on clear communication and a well-defined action plan. The insights gained from data analysis must be translated into concrete steps that various teams can execute. This often involves collaboration across departments and a commitment to tracking progress and making necessary adjustments along the way.
Several organizations have successfully leveraged strategic insights to achieve significant business outcomes:
- Netflix: By analyzing viewing habits and preferences, Netflix personalized recommendations, leading to increased user engagement and subscription retention. The insight: users prefer personalized content. The action: implementing a sophisticated recommendation algorithm.
- Amazon: Analyzing customer purchase data allowed Amazon to optimize its supply chain, leading to improved efficiency and reduced costs. The insight: predictive modeling of demand accurately forecasts sales. The action: Implementing AI-driven inventory management and logistics optimization.
- Starbucks: Analyzing customer loyalty program data helped Starbucks personalize offers and improve customer retention. The insight: rewarding frequent customers increases their spending and loyalty. The action: Targeted promotions and personalized offers based on purchase history.
Measuring the Impact of Strategic Insights
Unlocking the true value of strategic data insights isn’t just about gathering information; it’s about demonstrating a clear return on investment (ROI). Measuring the impact ensures that data-driven decisions are not only made but also proven to contribute meaningfully to the bottom line and overall business success. This involves a blend of quantitative and qualitative methods, providing a holistic view of the effectiveness of your strategic insights initiatives.Effective measurement requires a multifaceted approach.
We need to go beyond simply tracking immediate changes and instead focus on establishing a framework for long-term monitoring, allowing us to understand the ripple effects of our insights over time. This helps build a stronger case for future data-driven initiatives and fosters a culture of data-informed decision-making within the organization.
Quantitative Metrics for Evaluating Strategic Insights
Quantitative metrics provide concrete, measurable evidence of the impact of strategic insights. These metrics are crucial for demonstrating ROI and justifying continued investment in data-driven strategies. They allow for objective assessment and clear comparisons over time. For example, a marketing campaign fueled by customer segmentation insights might show a significant increase in conversion rates, measured as a percentage change from before the campaign.
Similarly, improvements in operational efficiency, such as reduced production costs or faster order fulfillment times, can be quantified and directly attributed to the application of data-driven insights. These numbers speak volumes and provide irrefutable evidence of success.
Qualitative Metrics for Evaluating Strategic Insights
While quantitative metrics offer hard numbers, qualitative metrics provide valuable context and deeper understanding. They explore the less easily measurable aspects of impact, such as improved employee satisfaction, enhanced customer experience, or increased brand loyalty. For instance, conducting post-implementation surveys to gauge employee satisfaction with new workflows optimized through data analysis provides valuable qualitative data. Similarly, analyzing customer feedback on a redesigned product based on market research insights helps assess the impact on customer perception and satisfaction.
These qualitative assessments enrich the overall picture, revealing the softer, yet equally important, benefits of strategic insights.
Framework for Tracking Long-Term Impact
A robust framework for tracking the long-term impact of strategic insights is essential. This framework should incorporate both quantitative and qualitative metrics, tracked consistently over time. Regular reporting, perhaps monthly or quarterly, is crucial to monitor progress and identify any deviations from projected outcomes. This ongoing monitoring allows for adjustments to strategies based on real-world performance data. Consider using a dashboard that visualizes key metrics, providing a clear and concise overview of the impact of implemented insights.
This allows for quick identification of areas needing attention and facilitates proactive adjustments to maintain momentum and achieve long-term objectives. For instance, tracking customer churn rate alongside customer satisfaction scores allows for a more comprehensive understanding of the long-term effects of a customer retention strategy implemented based on data-driven insights.
Challenges in Measuring ROI and Proposed Solutions
Measuring the ROI of strategic insights initiatives can present several challenges. Attributing specific outcomes solely to data-driven decisions can be difficult, especially when multiple factors influence business results. For example, separating the impact of a new marketing strategy from seasonal trends or competitor actions requires careful analysis and potentially advanced statistical methods. Another challenge lies in the difficulty of quantifying the impact of intangible benefits like improved brand reputation or increased employee morale.
To overcome these challenges, employ rigorous attribution modeling techniques that consider multiple factors influencing business outcomes. Qualitative data can help contextualize quantitative results, providing a more nuanced understanding of the overall impact. Finally, clearly defining success metrics upfront, linking them directly to business objectives, is crucial for accurate ROI measurement. This proactive approach sets the stage for effective evaluation from the outset.