Effective data visualization techniques for business intelligence dashboards are no longer a luxury but a necessity. In today’s data-driven world, turning raw numbers into compelling visuals is crucial for making sense of complex information and driving informed business decisions. This exploration delves into the art and science of crafting dashboards that not only present data but tell a story, revealing hidden trends and actionable insights.
Get ready to unlock the power of visual storytelling and transform your data into a strategic advantage.
We’ll cover a range of chart types, from classic bar graphs to more sophisticated heatmaps, and discuss when each is most effective. Beyond the charts themselves, we’ll dive into the crucial aspects of dashboard design, focusing on principles of clarity, usability, and effective color choices. Interactive elements, data storytelling techniques, and real-world case studies will further illuminate the path to creating truly impactful dashboards.
Finally, we’ll explore the various tools and technologies available to help you bring your data visualizations to life.
Introduction to Effective Data Visualization
Data visualization, in the context of business intelligence dashboards, is the art and science of translating complex data sets into easily understandable visual representations. It’s about transforming raw numbers and figures into compelling visuals that quickly communicate key insights, trends, and patterns. Effective data visualization isn’t just about making pretty pictures; it’s about facilitating clear, concise, and actionable understanding for better decision-making.The primary goals of using visualizations in business intelligence are multifaceted.
Ultimately, it aims to simplify complex data, revealing hidden patterns and relationships that might be missed in raw data analysis. This enhanced understanding empowers quicker and more informed decision-making, leading to improved business outcomes. Visualizations also improve communication; they make it easier to convey complex information to a wider audience, including stakeholders who may not have a strong analytical background.
Finally, effective visualizations help to identify areas needing further investigation, prompting deeper data exploration and potentially uncovering even more valuable insights.
Choosing the Right Visualization Type
Selecting the appropriate visualization type is crucial for effectively communicating data and answering specific business questions. The choice depends heavily on the type of data being analyzed and the insights sought. For instance, bar charts are ideal for comparing discrete categories, such as sales figures across different regions. Line charts excel at showing trends over time, like website traffic over a month.
Pie charts effectively illustrate proportions of a whole, such as market share distribution. Scatter plots are useful for identifying correlations between two variables, such as advertising spend and sales revenue. Choosing the wrong chart type can lead to misinterpretations and hinder effective decision-making. For example, using a pie chart to represent time-series data would be confusing and ineffective.
The key is to match the visualization to the data and the question being asked. Consider the type of data (categorical, numerical, temporal) and the nature of the comparison or relationship being investigated. A well-chosen chart clarifies complex information, while a poorly chosen one obscures it.
Chart Types for Business Intelligence Dashboards
Choosing the right chart type is crucial for effective data visualization in business intelligence dashboards. The goal is to present complex information clearly and concisely, allowing users to quickly grasp key insights and make informed decisions. The wrong chart can obscure data, leading to misinterpretations and poor business outcomes. This section explores various chart types and their suitability for different data scenarios.
Trend Visualization: Bar Charts, Line Charts, and Area Charts
Bar charts, line charts, and area charts are frequently used to display trends over time. However, each has its strengths and weaknesses. Bar charts are excellent for comparing discrete data points across different categories or time periods. They clearly show the magnitude of each data point, making it easy to identify highs and lows. Line charts, on the other hand, are best suited for showing continuous trends and highlighting changes over time.
The continuous line emphasizes the flow of data, making it easier to spot patterns and fluctuations. Area charts are similar to line charts but fill the area under the line, emphasizing the cumulative effect or total value over time. They are particularly useful when showing proportions or contributions to a total. For example, a bar chart might effectively show monthly sales figures for different product lines, while a line chart would be better for illustrating the growth of overall sales revenue over a year.
An area chart could then visualize the contribution of each product line to the overall revenue over time.
Comparison of Chart Types for Business Intelligence
The choice of chart type depends heavily on the data and the message you want to convey. Here’s a table comparing several common chart types:
Chart Type | Best Use Case | Data Suitability | Potential Drawbacks |
---|---|---|---|
Pie Chart | Showing proportions of a whole | Categorical data representing parts of a whole | Difficult to compare small segments, can be misleading with too many slices |
Bar Chart | Comparing categories or showing changes over time (discrete data) | Categorical or numerical data | Can become cluttered with many categories |
Line Chart | Showing trends and changes over time (continuous data) | Numerical data over time | Can be difficult to read with multiple lines |
Scatter Plot | Exploring relationships between two variables | Two numerical variables | Can be difficult to interpret with large datasets, doesn’t show causality |
Heatmap | Visualizing large datasets with color-coded values | Numerical data across multiple categories | Can be difficult to interpret with too many categories or values, colorblindness considerations |
Effective and Ineffective Uses of Pie Charts
Pie charts are effective when illustrating the proportion of different categories within a single whole. For instance, a pie chart clearly shows the market share of different competitors in a specific industry. However, they become less effective—and even misleading—when there are too many slices or when the differences between slices are minimal. An ineffective use would be attempting to show the sales figures of 10 different product lines with similar sales values in a single pie chart.
The numerous, similarly sized slices would make it nearly impossible to extract any meaningful information. A bar chart would be far more suitable in this case. Conversely, a pie chart showing the breakdown of a company’s revenue streams into three or four major categories (e.g., product sales, services, licensing) would be a clear and concise way to present this information.
Dashboard Design Principles for Effective Visualization: Effective Data Visualization Techniques For Business Intelligence Dashboards
Crafting compelling business intelligence dashboards isn’t just about choosing the right charts; it’s about designing an experience that seamlessly delivers insights. A well-designed dashboard prioritizes clarity, efficiency, and the effective communication of key performance indicators (KPIs). Poor design, on the other hand, can lead to confusion, misinterpretations, and ultimately, poor decision-making. This section explores key principles to guide you in creating dashboards that truly empower your business.Effective dashboard design hinges on several crucial elements that work together to create a powerful and easily digestible visualization of data.
These elements ensure that the information presented is not only accurate but also readily understood and actionable by the intended audience. Ignoring these principles can lead to cluttered, confusing dashboards that fail to deliver valuable insights.
Best Practices for Dashboard Design
A well-designed dashboard prioritizes ease of understanding and interpretation. This is achieved through a combination of strategic layout, consistent visual elements, and clear labeling. The following best practices ensure that your dashboards are effective tools for business intelligence.
- Prioritize Key Metrics: Focus on the most important KPIs and present them prominently. Less critical data can be relegated to secondary sections or made accessible through drill-downs.
- Maintain Visual Consistency: Use a consistent color palette, font styles, and chart types throughout the dashboard to maintain a cohesive and professional look. Inconsistency can distract the viewer and hinder understanding.
- Employ Clear and Concise Labels: All charts, graphs, and data points should be clearly labeled with concise and descriptive titles and axis labels. Avoid jargon and ensure that labels are easily readable.
- Use White Space Effectively: White space, or the empty areas around elements, is crucial for improving readability and preventing a cluttered appearance. Don’t overcrowd the dashboard.
- Ensure Accessibility: Design the dashboard to be accessible to users with disabilities, considering factors like color contrast, font size, and keyboard navigation.
Effective Use of Color in Dashboards
Color plays a vital role in highlighting key insights and guiding the viewer’s attention. However, improper use of color can lead to misinterpretations and even distort the data.The strategic use of color can significantly enhance the effectiveness of a dashboard. For example, using a consistent color scheme to represent specific data categories creates visual cohesion and simplifies data interpretation.
A well-defined color palette helps to highlight key trends and outliers, guiding the viewer’s eye to the most important information. Conversely, overuse of colors or the use of clashing colors can create a confusing and visually unappealing dashboard, making it difficult to extract meaningful insights.Consider these guidelines:
- Use a Limited Color Palette: Stick to a small number of colors to avoid overwhelming the viewer. A maximum of three to five colors is generally recommended.
- Use Color Strategically: Employ color to highlight key data points, trends, or outliers. Use contrasting colors to draw attention to critical information.
- Consider Color Blindness: Choose colors that are easily distinguishable by individuals with color blindness. Avoid using red and green together, for example.
- Maintain Consistency: Use the same color to represent the same data point or category throughout the dashboard.
Designing a Dashboard Layout: Prioritizing Information
Designing a dashboard layout that prioritizes the most important information requires a systematic approach. This ensures that critical insights are immediately apparent to the user.A step-by-step guide to designing an effective dashboard layout involves a clear understanding of the target audience and their information needs. The design should then reflect this understanding by prioritizing the most critical information upfront.
This might involve using a hierarchical structure, where the most important KPIs are presented prominently at the top of the dashboard, followed by supporting information in subsequent sections. This hierarchical structure aids in quickly conveying the most relevant information while allowing for a deeper dive into more detailed data when needed.
- Identify Key Performance Indicators (KPIs): Begin by identifying the most important KPIs that need to be tracked and displayed.
- Determine the Target Audience: Understand the needs and information requirements of the users who will be interacting with the dashboard.
- Choose Appropriate Chart Types: Select the chart types that best represent the data and are easily understood by the target audience.
- Create a Wireframe: Sketch a basic layout of the dashboard, placing the most important KPIs in the most prominent locations.
- Iterate and Refine: Test the dashboard with users and refine the design based on their feedback.
Interactive Elements and User Experience
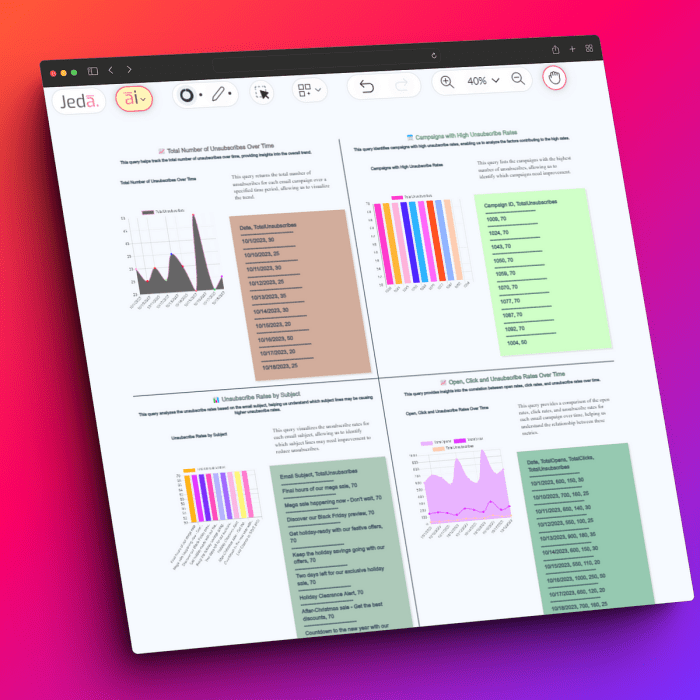
Interactive dashboards are no longer a luxury; they’re a necessity for effective business intelligence. Static visualizations simply can’t keep up with the need for agile data exploration and insightful decision-making in today’s fast-paced business world. By incorporating interactive elements, we empower users to delve deeper into the data, uncovering hidden patterns and gaining a more comprehensive understanding of their business performance.Adding interactivity significantly enhances the user experience, transforming a passive viewing experience into an active exploration.
Users can manipulate the data, focusing on specific aspects that are most relevant to their immediate needs. This empowers them to answer their own questions, leading to more informed and timely decisions. Think of it as giving your users a powerful magnifying glass to zoom in on the details that matter most, rather than just presenting a static overview.
Interactive Dashboard Element Example
Consider a sales dashboard displaying regional sales performance. A simple bar chart shows total sales for each region. However, adding interactivity transforms this static view into a dynamic tool. Imagine clicking on a specific bar representing a region, triggering a drill-down to a more granular view showing sales broken down by product category within that region. Further interaction could allow filtering by time period (e.g., month, quarter, year) or by individual sales representatives.This functionality could be implemented using HTML, JavaScript, and a charting library like D3.js or Chart.js.
For instance, each bar could be represented as a `
` element with an event listener attached. When clicked, the event listener would trigger an AJAX request to fetch the more detailed data, which would then be used to update the chart or display a separate chart. The underlying data could be stored in a JSON format, making it easy to access and manipulate. This example demonstrates a simple drill-down; more complex interactions, such as linked brushing and filtering across multiple charts, are also possible.
Improving User Experience Through Design Considerations
Effective dashboard design is crucial for a positive user experience. Data density needs careful consideration. Too much information overwhelms users; too little leaves them wanting more. A balanced approach is key, prioritizing the most important metrics and presenting them clearly. Visual hierarchy guides the user’s eye, leading them to the most critical information first.
This is achieved through the strategic use of size, color, and position. For example, key performance indicators (KPIs) should be prominently displayed, while less important details can be relegated to secondary positions.Accessibility is paramount. Dashboards must be usable by everyone, regardless of visual or other impairments. This requires adherence to accessibility guidelines, such as providing alternative text for images and ensuring sufficient color contrast.
Consider using clear and concise labels, avoiding jargon, and offering various data formats (e.g., tables, charts) to cater to diverse user preferences and needs. For example, colorblind users may need alternative ways to distinguish data points, such as different patterns or shapes. Proper use of ARIA attributes can enhance accessibility for screen reader users.
Effective data visualization in business intelligence dashboards relies on clear, concise representations of key metrics. Choosing the right charts and graphs is crucial for insightful decision-making, and a robust ERP system like acumatica erp can significantly improve data accessibility. Ultimately, leveraging powerful visualization techniques alongside a streamlined ERP enhances the overall effectiveness of your business intelligence strategy.
Data Storytelling with Visualizations
Data visualization isn’t just about presenting data; it’s about crafting a narrative that resonates with your audience and drives informed business decisions. Effective data storytelling uses visuals to communicate complex information clearly and persuasively, transforming raw numbers into compelling insights. By guiding the viewer through a logical sequence of visualizations, you can build a story that supports your key findings and ultimately influences business strategies.Data visualization allows you to translate complex datasets into easily digestible narratives.
Instead of overwhelming your audience with spreadsheets or dense reports, you can leverage charts and graphs to highlight trends, patterns, and outliers, making the information more accessible and memorable. This approach is crucial in business intelligence, where quick comprehension and actionable insights are paramount. A well-crafted visual story can significantly improve decision-making by making the data’s implications immediately clear.
Visual Storytelling Techniques
Effective visual storytelling involves strategically employing various techniques to guide the viewer’s eye and emphasize critical information. Annotations, labels, and summaries play crucial roles in providing context and clarifying complex data points. For instance, an annotated line chart showing sales trends could highlight specific periods of growth or decline with text boxes, contextualizing the data fluctuations and making them easier to understand.
Similarly, clear and concise labels on charts ensure data points are immediately identifiable, while strategically placed summaries provide concise overviews of key findings.
Guiding User Attention with Visual Cues
Directing the user’s attention to crucial insights is paramount in effective data storytelling. This can be achieved through a variety of visual cues. Using color strategically, for example, can highlight key data points or trends. A heatmap might use a gradient to show the intensity of a particular metric, immediately drawing the eye to the areas of highest or lowest values.
Similarly, size and shape can be used effectively. Larger bars in a bar chart automatically attract attention, highlighting significant differences between categories. Animation, when used judiciously, can further guide the viewer’s eye through the narrative, emphasizing transitions and changes over time. Consider a chart animating the growth of a company’s market share year over year; the animation naturally draws attention to the growth trajectory.
Examples of Effective Visual Storytelling
Imagine a dashboard showing the performance of different marketing campaigns. Instead of simply presenting raw numbers for click-through rates, conversion rates, and cost-per-acquisition, a compelling narrative could be built. A series of interconnected charts could visually demonstrate the relationship between marketing spend and return on investment for each campaign. Annotations could highlight the most successful campaign and explain the reasons for its success, while a summary table could quickly compare the key performance indicators (KPIs) across all campaigns.
This approach transforms a collection of data points into a clear, concise story that informs strategic decisions about future marketing investments. Another example could involve visualizing customer churn rate over time. A line graph showing a consistent downward trend, accompanied by annotations explaining implemented strategies, paints a picture of success and demonstrates the impact of specific interventions.
Case Studies of Effective Visualizations
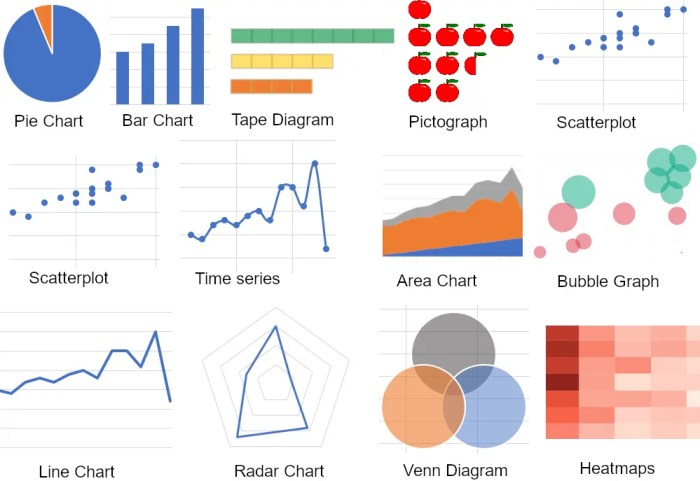
Data visualization isn’t just about pretty charts; it’s about translating complex data into actionable insights. Effective visualizations can dramatically improve decision-making, boost efficiency, and ultimately drive business success. Let’s examine some real-world examples that showcase the power of well-designed dashboards.
Case Study 1: Netflix’s Content Recommendation System
Netflix faced the challenge of personalizing its vast content library to keep subscribers engaged. Their solution involved a sophisticated data visualization system that analyzed viewing habits, ratings, and other user data to recommend relevant movies and shows. This system utilized a combination of visualizations. Heatmaps illustrated viewing patterns across different demographics and time periods, highlighting popular content and potential gaps.
Interactive network graphs visualized relationships between different movies and shows, based on viewer preferences, allowing Netflix to identify potential clusters of related content for recommendations. The result? Improved user engagement, increased subscription retention, and a significant boost in revenue driven by targeted recommendations. The key visualization elements were interactive, allowing users to explore the data dynamically. The system continuously evolved, adapting to changing viewer preferences.
Case Study 2: Walmart’s Supply Chain Optimization
Walmart, a retail giant, needed to optimize its vast and complex supply chain to minimize costs and improve efficiency. They leveraged data visualization to gain real-time insights into inventory levels, transportation routes, and demand forecasting. Dashboards displayed key performance indicators (KPIs) such as on-time delivery rates, inventory turnover, and transportation costs using bar charts, line graphs, and geographical maps.
These visuals allowed Walmart to identify bottlenecks and inefficiencies in their supply chain, enabling them to make data-driven decisions to improve logistics, reduce waste, and ultimately lower operational costs. A crucial aspect of their success was the ability to drill down into the data, exploring specific regions or product categories to uncover granular details. The visualizations were designed to be easily understandable by managers at all levels, facilitating collaboration and informed decision-making.
Case Study 3: The New York Times’ Election Coverage, Effective data visualization techniques for business intelligence dashboards
The New York Times used data visualization extensively during the 2020 US Presidential Election to present complex election data in an accessible and engaging manner. They employed a variety of visualizations, including interactive maps showing real-time vote counts, charts illustrating key demographic trends, and graphs comparing different candidates’ performance in various regions. These visualizations were not only informative but also visually appealing, making complex data easy to understand for a broad audience.
The interactive nature of the maps and charts allowed users to explore the data at different levels of detail, making it an engaging and informative experience. The result was increased user engagement and a deeper understanding of the election results for their readership. This case highlights the importance of clear, concise, and aesthetically pleasing visualizations in conveying complex information effectively to a wide audience.
Common Elements Contributing to Success
These case studies demonstrate several common elements crucial for effective data visualization: interactivity, clear and concise visuals, focus on key performance indicators (KPIs), and the ability to drill down into the data for deeper analysis. Furthermore, tailoring the visualization to the specific audience and their needs is paramount.
Limitations and Challenges
Despite the benefits, challenges exist. Data quality and accuracy are crucial. Inaccurate data will lead to misleading visualizations. Moreover, the design and implementation of effective dashboards require expertise in data visualization, data analysis, and user experience design. Finally, maintaining and updating dashboards requires ongoing effort to ensure the data remains relevant and the visualizations are effective.
Tools and Technologies for Data Visualization
Choosing the right tools for data visualization is crucial for creating effective business intelligence dashboards. The ideal tool depends on factors like data volume, complexity, desired interactivity, technical expertise within the team, and budget constraints. Different tools cater to different skill sets and project needs, ranging from user-friendly drag-and-drop interfaces to powerful programming libraries.The selection process often involves comparing features, ease of use, integration capabilities, and cost-effectiveness.
Understanding the strengths and weaknesses of various tools allows businesses to optimize their data visualization workflow and maximize the impact of their dashboards.
Data Visualization Tool Comparison
Several popular tools dominate the data visualization landscape, each offering unique capabilities. Tableau and Power BI, for instance, are known for their user-friendly interfaces and robust features, while tools like Qlik Sense excel in associative data exploration. On the other hand, programming languages like Python (with libraries like Matplotlib, Seaborn, and Plotly) and R (with ggplot2 and others) provide greater control and customization but require more technical expertise.
Tool | Strengths | Weaknesses | Suitable for |
---|---|---|---|
Tableau | Intuitive drag-and-drop interface, strong data connectivity, excellent visualization options, robust community support. | Can be expensive, steep learning curve for advanced features. | Users with varying technical skills; ideal for quick prototyping and interactive dashboards. |
Power BI | Excellent integration with Microsoft ecosystem, affordable, strong data modeling capabilities, good for large datasets. | Limited customization options compared to some other tools. | Businesses heavily invested in the Microsoft ecosystem; suitable for creating interactive reports and dashboards. |
Qlik Sense | Associative data exploration, strong data discovery capabilities, good for uncovering hidden insights. | Steeper learning curve than Tableau or Power BI. | Users who need to explore complex datasets and uncover unexpected relationships. |
Python (with Matplotlib, Seaborn, Plotly) | Highly customizable, open-source, large community support, powerful for data manipulation and analysis. | Requires programming skills, steeper learning curve. | Users with strong programming skills; ideal for creating highly customized and complex visualizations. |
R (with ggplot2) | Excellent for statistical analysis and visualization, open-source, vast library of packages. | Steeper learning curve than Python, less intuitive interface. | Users with strong statistical background and programming skills; excellent for creating publication-quality graphics. |
Handling Different Data Types and Visualization Needs
Different tools handle various data types and visualization needs with varying degrees of efficiency. For example, Tableau excels at handling large datasets and creating interactive maps, while Power BI is strong in integrating data from various sources, including Excel spreadsheets and SQL databases. Python, with its extensive libraries, offers unparalleled flexibility in handling diverse data formats and creating custom visualizations, from simple bar charts to complex network graphs.
For instance, a business analyzing sales data across different regions might find Tableau’s mapping capabilities beneficial, while a business analyzing complex financial data might prefer the customization options offered by Python.
Advantages and Disadvantages of Software and Programming Languages
Using dedicated data visualization software like Tableau or Power BI offers advantages such as ease of use, pre-built visualizations, and strong community support. However, they might lack the flexibility and customization options available with programming languages like Python or R. Programming languages, on the other hand, provide maximum control and allow for the creation of highly customized visualizations, but they require a higher level of technical expertise and a steeper learning curve.
The choice often comes down to balancing the need for speed and ease of use with the need for highly specific and tailored visualizations. For instance, a small business might find Tableau sufficient, while a large corporation with complex data analysis needs might opt for a combination of Power BI and Python for different tasks.