Business iintelligence segments – Business intelligence segments are the backbone of effective data-driven decision-making. Understanding how to segment your business, collect and analyze the relevant data, and then translate those findings into actionable strategies is crucial for growth and competitive advantage. This deep dive explores the entire process, from defining your segments to visualizing the results in impactful dashboards.
We’ll cover various segmentation strategies, including demographic, geographic, and behavioral approaches, and examine the best methods for collecting, integrating, and analyzing data across these segments. Learn how to identify key performance indicators (KPIs), interpret the results, and ultimately use this information to refine your customer targeting, boost operational efficiency, and make smarter, more informed business decisions.
Defining Business Intelligence Segments
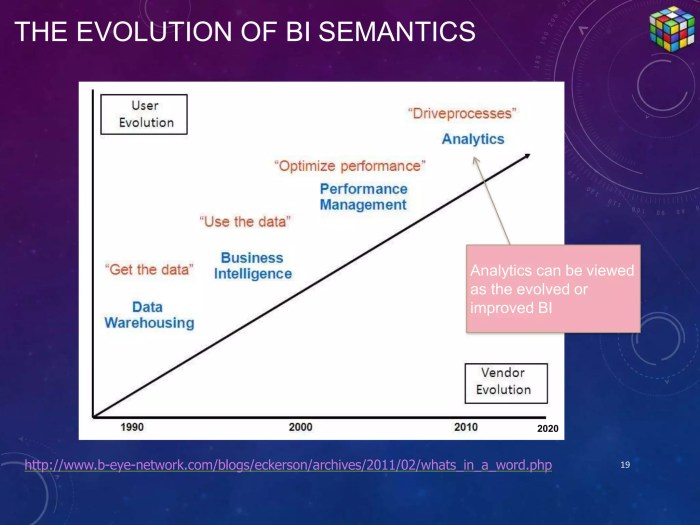
Unlocking the power of Business Intelligence (BI) hinges on effectively segmenting your data. Without a clear understanding of your customer base and operational intricacies, your BI efforts will yield limited insights. Effective segmentation allows businesses to tailor strategies, optimize operations, and ultimately, boost profitability. This involves dividing your data into meaningful groups based on shared characteristics.
Different Ways to Segment Businesses for BI Purposes
Businesses can be segmented in numerous ways, depending on their objectives and the data available. The key is to choose a segmentation strategy that aligns with your specific business goals and provides actionable insights. This allows for a more focused and efficient use of resources, maximizing the return on investment in your BI initiatives. For example, a retail company might segment its customers based on purchasing behavior, while a manufacturing firm might segment its production lines based on efficiency metrics.
Examples of Common Segmentation Strategies
Several common strategies effectively segment data for BI purposes. Demographic segmentation, for instance, utilizes readily available data points like age, gender, income, and location. Geographic segmentation focuses on location-based factors, such as region, country, or even specific zip codes. Behavioral segmentation delves into customer actions, including purchasing history, website activity, and engagement with marketing campaigns. For example, a clothing retailer might segment its customers based on their preferred clothing styles (e.g., casual, formal), while a bank might segment its customers based on their risk profiles and investment habits.
A food delivery service might segment its customers based on their order frequency and average order value.
Criteria for Selecting Appropriate Segmentation Methods, Business iintelligence segments
Choosing the right segmentation method is crucial. The selection process should consider several key factors. First, clearly define your business objectives. What questions are you trying to answer with your BI analysis? Second, evaluate the availability and quality of your data.
Do you have the necessary data to support your chosen segmentation method? Third, consider the feasibility and cost of implementing your chosen method. Some methods require more sophisticated data analysis techniques than others. Finally, ensure your chosen method aligns with your overall BI strategy and contributes to your business objectives. For example, a small business with limited data might opt for a simpler demographic segmentation approach, while a large multinational corporation might employ a more complex multi-faceted segmentation strategy.
Comparison of Different Segmentation Techniques
Segmentation Technique | Advantages | Disadvantages | Example |
---|---|---|---|
Demographic | Easy to understand and implement; readily available data | May not capture nuanced customer behaviors; can lead to overgeneralizations | Segmenting customers based on age and income to target specific marketing campaigns. |
Geographic | Useful for location-based businesses; allows for targeted marketing campaigns | May not be relevant for businesses with a global reach; can be too broad | Segmenting customers based on region to tailor product offerings to local preferences. |
Behavioral | Provides insights into customer actions and preferences; allows for personalized experiences | Requires more sophisticated data analysis techniques; data privacy concerns | Segmenting customers based on their website browsing history to recommend relevant products. |
Psychographic | Provides a deeper understanding of customer motivations and values; allows for more targeted marketing | Data collection can be challenging; requires advanced analytical techniques | Segmenting customers based on their lifestyle and interests to create more engaging marketing campaigns. |
Data Collection and Integration for Segmented BI
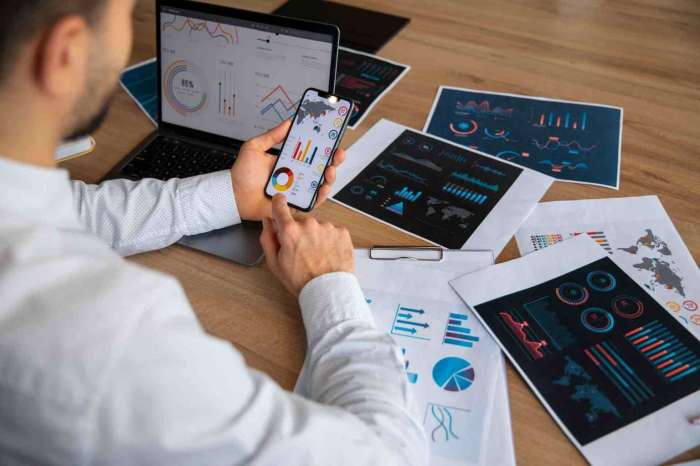
Unlocking the true power of Business Intelligence (BI) lies in its ability to deliver tailored insights to specific segments within your organization. This requires a robust and efficient system for collecting, integrating, and preparing data relevant to each segment. Failing to do so results in a fragmented view of your business, hindering strategic decision-making. This section dives into the crucial processes involved in achieving a unified and actionable data landscape for segmented BI.Data collection methods must align with the unique characteristics of each segment.
For example, the data needed to understand customer segmentation based on demographics will differ significantly from the data required to analyze sales performance by product category. Effective data collection hinges on understanding these segment-specific needs.
Data Collection Methods for Segmented BI
Choosing the right data collection methods is paramount for accuracy and efficiency. Different segments may necessitate various approaches. For instance, customer segmentation might utilize CRM data, website analytics, and survey responses. Meanwhile, analyzing operational efficiency might rely on ERP systems, production logs, and internal performance metrics. A diverse strategy ensures comprehensive data capture.
Ensuring Data Quality and Consistency Across Segments
Data quality is the cornerstone of reliable BI. Inconsistent or inaccurate data can lead to flawed analyses and poor decision-making. Establishing robust data governance procedures is crucial. This includes implementing data validation rules, regularly auditing data sources, and employing data cleansing techniques to identify and correct errors. Furthermore, standardizing data formats and definitions across all segments is vital for ensuring consistency and comparability.
For example, using a consistent format for date and time entries across all data sources prevents discrepancies in reporting.
Data Integration Techniques for Segmented BI
Integrating data from various sources—CRMs, ERPs, marketing automation platforms, and more—is a complex but essential task. Modern ETL (Extract, Transform, Load) processes and data integration platforms play a critical role. These tools allow for the efficient extraction of data from disparate sources, transformation into a standardized format, and loading into a central data warehouse or data lake. This unified view facilitates cross-segment analysis and provides a holistic understanding of the business.
Techniques like data mapping and schema design are crucial for ensuring data integrity during the integration process. For example, mapping customer IDs from the CRM to transaction IDs in the sales database creates a unified customer profile.
Workflow Diagram for Segmented BI Data Processing
Imagine a flowchart. The process begins with Data Sources (represented by boxes labeled “CRM,” “ERP,” “Website Analytics,” etc.). Arrows flow from these boxes to a central Data Integration Hub (a large oval). Inside this hub, processes like Data Cleaning, Transformation, and Validation are depicted (smaller ovals within the large oval). Arrows then lead from the Data Integration Hub to a Data Warehouse/Lake (a large rectangular box).
Finally, arrows extend from the Data Warehouse/Lake to BI Dashboards and Reports (smaller rectangular boxes representing various segment-specific reports). This illustrates the flow of data from diverse sources to a unified, prepared dataset ready for analysis. The entire process is cyclical, with regular data updates and refinement.
Analyzing Data Within Segments
Unlocking the true power of Business Intelligence lies not just in collecting data, but in skillfully analyzing it to reveal actionable insights. Segmented BI allows us to drill down into specific customer groups, product lines, or geographic regions, providing a far more granular understanding of performance than traditional, aggregate analysis. By analyzing data within these pre-defined segments, businesses can identify areas for improvement, optimize strategies, and ultimately, drive growth.Analyzing data within segments involves a systematic process of identifying key performance indicators (KPIs), comparing segment performance, and presenting findings in a clear, easily digestible format.
This approach allows for a deeper understanding of what’s working and what needs attention, paving the way for data-driven decision-making.
Key Performance Indicators (KPIs) for Segment Analysis
Choosing the right KPIs is crucial for effective segment analysis. The specific KPIs will vary depending on the business goals and the nature of the segments. For example, an e-commerce business might track conversion rates, average order value, and customer lifetime value for different customer segments based on demographics or purchase history. A manufacturing company might focus on production efficiency, defect rates, and machine downtime for different product lines.
Selecting the appropriate KPIs ensures that the analysis directly addresses the most important business questions.
Comparing Segment Performance
Once KPIs are identified, the next step involves comparing the performance of different segments. This comparison reveals variations in performance across the segments and highlights areas of strength and weakness. For instance, a comparison might reveal that one customer segment has a significantly higher conversion rate than others, indicating a successful marketing strategy targeted at that specific group. Conversely, a low conversion rate in another segment might signal the need for adjustments to the marketing approach or product offerings.
This comparative analysis provides a clear picture of which segments are performing well and which require attention.
Reporting Analysis Results
Presenting the analysis results clearly and concisely is essential for effective communication. This often involves using charts and graphs to visually represent the data. For example, a bar chart could effectively compare the average order value across different customer segments. The chart would show distinct bars for each segment, with the height of each bar representing the average order value.
A visually striking difference in bar heights would immediately highlight segments with significantly higher or lower average order values. Similarly, a line graph could track the conversion rate over time for each segment, showing trends and patterns in performance. This graph would display multiple lines, each representing a segment’s conversion rate over the chosen period. The slope and overall trajectory of each line would indicate the performance trend for that particular segment.
Accompanying these visuals, descriptive text should explain the key findings and implications of the data.
Interpreting Segmented Data Analysis Findings
Interpreting the findings requires a critical understanding of the context surrounding the data. For example, a high conversion rate in one segment might be attributed to a successful marketing campaign targeted specifically at that group. Conversely, a low conversion rate in another segment might suggest a need for product improvements or a reevaluation of the marketing strategy. It’s crucial to consider external factors, such as seasonal variations or economic conditions, when interpreting the results.
The analysis should provide actionable insights, guiding decisions on resource allocation, marketing strategies, product development, and other business operations. For example, if analysis reveals a segment with consistently high customer lifetime value, the company might invest more resources in retaining and nurturing customers within that segment.
Actionable Insights and Strategic Implications: Business Iintelligence Segments
Segmented business intelligence doesn’t just provide data; it unlocks strategic advantages. By analyzing data sliced by specific customer groups, product lines, or geographic regions, businesses gain a granular understanding of their operations and market position, leading to more effective decision-making and improved outcomes. This section explores how actionable insights derived from segmented BI can drive significant improvements across various aspects of a business.Analyzing segmented data reveals opportunities for targeted interventions and strategic adjustments.
This allows businesses to move beyond broad strokes and focus resources where they will have the most impact. For example, identifying a segment with high churn rates allows for proactive interventions, potentially retaining valuable customers and mitigating revenue loss. Conversely, identifying a high-growth segment enables businesses to optimize resource allocation to maximize expansion potential.
Improved Customer Targeting and Retention
Understanding customer segments allows for highly targeted marketing campaigns. For example, a clothing retailer might identify a segment of young, fashion-conscious consumers who respond well to social media advertising. By tailoring marketing messages and channels to this specific segment, the retailer can maximize its return on investment (ROI) compared to a broad-based campaign. Similarly, identifying segments with low purchase frequency can trigger personalized retention strategies, such as loyalty programs or exclusive offers, aimed at increasing engagement and lifetime value.
This precision targeting minimizes wasted resources and maximizes campaign effectiveness.
Enhanced Operational Efficiency and Resource Allocation
Segmented BI offers a clear view of operational bottlenecks and inefficiencies. Consider a manufacturing company analyzing production data segmented by product line. Identifying a specific product line with consistently high defect rates allows the company to pinpoint problems in the production process, optimize quality control measures, and potentially reduce waste. Similarly, analyzing sales data segmented by region can reveal underperforming areas, prompting targeted sales initiatives or adjustments to distribution strategies.
This data-driven approach optimizes resource allocation, improving overall efficiency and profitability.
Specific Actions Based on Segmented Insights
The insights gained from segmented BI translate directly into actionable steps. The specific actions will vary depending on the business and the segments identified, but here are some examples:
- For a segment showing high churn: Implement a targeted customer retention program offering exclusive discounts, personalized communication, or proactive support to address specific pain points identified within the segment.
- For a high-growth segment: Increase investment in marketing and sales efforts targeted at this segment, expand product offerings to meet their specific needs, and explore new market expansion opportunities.
- For a segment with low purchase frequency: Develop a loyalty program offering rewards for repeat purchases, personalized recommendations, or exclusive early access to new products.
- For a segment exhibiting high customer satisfaction: Identify the key factors driving satisfaction and replicate them across other segments. This can involve improving customer service protocols or refining product features.
- For a segment with consistently low conversion rates: Analyze the customer journey within this segment to identify friction points. This might involve redesigning the website, simplifying the purchasing process, or improving product descriptions.
Visualizing Segmented BI Data
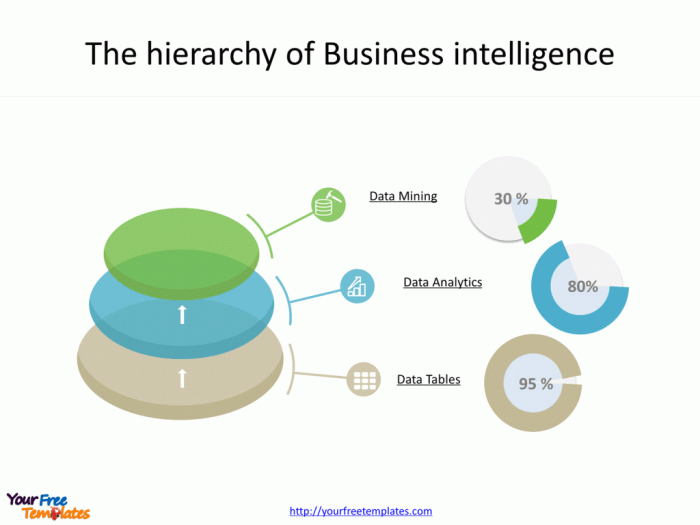
Data visualization is the cornerstone of effective Business Intelligence. Turning raw, segmented data into compelling visuals allows for quick comprehension and informed decision-making. By strategically representing key performance indicators (KPIs) for each segment, businesses can identify trends, outliers, and opportunities for improvement with unprecedented clarity. This section explores the art and science of visualizing segmented BI data, focusing on dashboard design, visualization techniques, and audience considerations.Interactive dashboards are crucial for navigating the complexities of segmented data.
They provide a dynamic and engaging way to explore KPIs across different segments, enabling users to drill down into details and uncover hidden insights. Effective dashboards combine clear layouts with intuitive interactive elements, facilitating seamless data exploration and analysis.
Interactive Dashboard Design
A well-designed interactive dashboard for segmented BI should prioritize clarity and ease of use. The layout should be logical and intuitive, using color-coding and visual hierarchies to guide the user’s eye. Interactive elements, such as filters, drill-downs, and tooltips, empower users to explore data at different levels of granularity. Data representation techniques should be chosen based on the type of data and the insights being communicated.
For example, line charts are ideal for showing trends over time, while bar charts are better for comparing values across different segments. Pie charts can illustrate proportions, and scatter plots can reveal correlations between variables. The dashboard should be responsive, adapting seamlessly to different screen sizes and devices. Consider a layout where key KPIs are prominently displayed, followed by more detailed views accessible through interactive elements.
For instance, a summary section could display overall performance metrics for all segments, while individual sections could provide a deeper dive into each segment’s performance.
Effective Data Visualization Techniques
The choice of visualization technique should align with the specific needs of each segment and the type of data being presented. For example, a segment focused on customer retention might benefit from a line chart showing customer churn over time, while a segment focused on sales might use a geographical heatmap to identify high-performing regions. For a segment focused on marketing campaign performance, a bar chart comparing the effectiveness of different campaigns would be suitable.
A segment analyzing website traffic might find value in funnel charts to visualize user journeys. Choosing the right visualization ensures the data is presented in a clear, concise, and easily understandable manner.
Audience Considerations in Visualization
The audience for the visualizations significantly influences the choice of charts and the level of detail included. Executive dashboards should focus on high-level summaries and key takeaways, while operational dashboards might require more granular data and detailed breakdowns. Technical audiences may appreciate complex visualizations and detailed data points, while non-technical audiences might benefit from simpler, more intuitive representations.
Consider the level of data literacy of the audience when choosing visualizations. Avoid using overly complex or obscure charts that may confuse or overwhelm the audience.
Heatmap Example: Segment Performance Across KPIs
A heatmap is an excellent visualization technique for showing the performance of various segments across different KPIs. Imagine a heatmap where each row represents a customer segment (e.g., high-value customers, new customers, loyal customers), and each column represents a KPI (e.g., customer lifetime value (CLTV), average order value (AOV), customer churn rate). Each cell in the heatmap is color-coded to represent the performance of a specific segment on a specific KPI.
Darker shades of green might indicate high performance, while darker shades of red might indicate low performance. This visualization allows for a quick comparison of segment performance across multiple KPIs, facilitating the identification of high-performing and underperforming segments. For instance, a dark green cell in the “high-value customers” row and “CLTV” column would indicate that high-value customers have a high CLTV, while a dark red cell in the “new customers” row and “CLTV” column would indicate that new customers have a low CLTV.
This provides a clear and concise overview of the performance of different customer segments across various key metrics.