Business intelligence solutions for improving operational decision-making are revolutionizing how businesses operate. By harnessing the power of data, companies can gain unprecedented insights into their operations, leading to more efficient processes, smarter strategies, and ultimately, a significant boost to the bottom line. This deep dive explores how real-time data, advanced analytics, and intuitive dashboards are transforming decision-making across various industries, from streamlining supply chains to optimizing customer service.
We’ll uncover the key components of a robust BI system, examine various data sources and integration challenges, and delve into the specific tools and technologies that empower businesses to make data-driven decisions. From choosing the right BI platform to measuring the ROI of your investment, we’ll equip you with the knowledge to leverage BI for impactful operational improvements.
Defining Business Intelligence (BI) for Operational Efficiency
Business Intelligence (BI) isn’t just about crunching numbers; it’s about transforming raw data into actionable insights that drive operational efficiency. A robust BI system empowers businesses to make smarter, faster decisions, ultimately boosting productivity and profitability. Think of it as your company’s strategic compass, guiding you towards optimal performance.
Core Components of a Robust BI System for Operational Improvements
A truly effective BI system for operational improvements requires a synergistic blend of several key components. These components work together to collect, process, analyze, and visualize data, ultimately providing a clear picture of operational performance. Without a solid foundation in each area, the system’s effectiveness is severely hampered.
Real-time Data Integration for Enhanced Operational Decision-Making
Real-time data integration is the lifeblood of a responsive and efficient BI system. Imagine a scenario where sales data is updated instantly, allowing you to adjust inventory levels immediately or even personalize marketing campaigns in real-time based on current customer behavior. This immediate access to information allows for proactive adjustments, preventing potential problems before they escalate and maximizing opportunities as they arise.
For example, a retail chain could use real-time sales data to identify high-demand products, instantly adjusting stock levels in specific locations to prevent stockouts and maximize sales. This agility is impossible with delayed or batch-processed data.
Data Visualization Tools for Operational Insights
Data visualization is the art of transforming complex data into easily understandable visual representations. Instead of wading through endless spreadsheets, BI dashboards provide a clear, concise overview of key performance indicators (KPIs). Think interactive charts, graphs, and maps that highlight trends, patterns, and anomalies. This visual approach makes complex data accessible to everyone, from executives to frontline employees, fostering a data-driven culture within the organization.
For instance, a heatmap visualizing customer complaints by geographic location can quickly identify areas needing immediate attention, leading to targeted improvements in customer service.
Key Performance Indicators (KPIs) for Operational Efficiency
Tracking the right KPIs is crucial for measuring and improving operational efficiency. A BI system should track metrics directly related to operational goals. These could include things like: On-time delivery rates, Production cycle times, Inventory turnover, Customer service response times, Defect rates, and Employee productivity. For example, consistently tracking on-time delivery rates can highlight bottlenecks in the supply chain, allowing for targeted improvements in logistics and scheduling.
By monitoring these KPIs, businesses gain a clear understanding of their operational strengths and weaknesses, paving the way for targeted improvements.
Data Sources and Integration for Operational BI
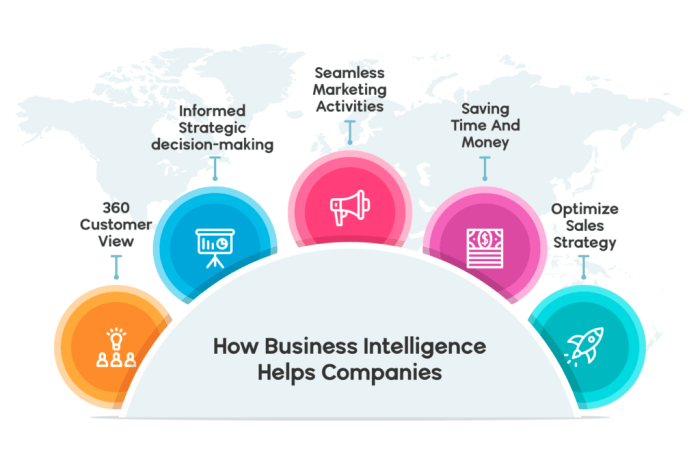
Operational Business Intelligence (BI) thrives on the ability to gather, integrate, and analyze data from various sources to drive timely and effective decision-making. The richer and more diverse the data sources, the more comprehensive and insightful the operational intelligence becomes. However, this richness also presents significant integration challenges.
Data Sources for Operational BI
Operational BI draws data from a multitude of sources, each offering a unique perspective on business operations. These sources often include internal systems like Enterprise Resource Planning (ERP) systems, Customer Relationship Management (CRM) systems, and Supply Chain Management (SCM) systems. Beyond these, external data sources like market research reports, social media sentiment analysis, and Internet of Things (IoT) devices contribute valuable real-time insights.
ERP systems provide data on production, inventory, and finance, while CRM systems offer customer interaction details. SCM systems track logistics and supply chain performance, and IoT devices provide real-time sensor data on equipment and environmental conditions. The integration of these disparate data sources is crucial for a holistic view of operational efficiency.
Challenges of Data Integration in Operational BI
Integrating data from diverse sources is rarely straightforward. Data inconsistencies, varying formats, and differing data structures pose significant hurdles. Data silos, where data is isolated within different systems, further complicate the process. For example, a discrepancy in product codes between the ERP and CRM systems can lead to inaccurate sales reporting. Differences in data formats (e.g., date formats, currency symbols) also necessitate careful transformation before integration.
Furthermore, security and access control issues must be addressed to ensure data privacy and compliance.
Data Cleaning and Transformation Processes
Before data can be effectively analyzed, it must undergo rigorous cleaning and transformation. Data cleaning involves identifying and correcting inconsistencies, errors, and inaccuracies. This may include handling missing values, removing duplicates, and standardizing data formats. Data transformation involves converting data into a suitable format for analysis. This could include aggregating data, creating new variables, and normalizing data to a consistent scale.
For instance, transforming raw sales data into weekly or monthly sales figures allows for trend analysis. Robust data quality checks and validation procedures are essential to ensure the accuracy and reliability of the final dataset.
Data Pipeline Architecture for Operational BI
A well-designed data pipeline is essential for efficient data flow within an operational BI system. This pipeline typically involves several stages, from data extraction to data visualization. The following table Artikels a sample architecture:
Step | Tools | Responsibilities |
---|---|---|
Data Extraction | ETL tools (e.g., Informatica, Talend), APIs | Data engineers, IT team |
Data Transformation | ETL tools, scripting languages (e.g., Python, R) | Data engineers, data scientists |
Data Loading | Data warehouses (e.g., Snowflake, BigQuery), data lakes | Data engineers, database administrators |
Data Modeling | Data modeling tools, SQL | Data architects, data engineers |
Data Visualization & Reporting | BI tools (e.g., Tableau, Power BI) | Data analysts, business users |
BI Tools and Technologies for Operational Decisions: Business Intelligence Solutions For Improving Operational Decision-making
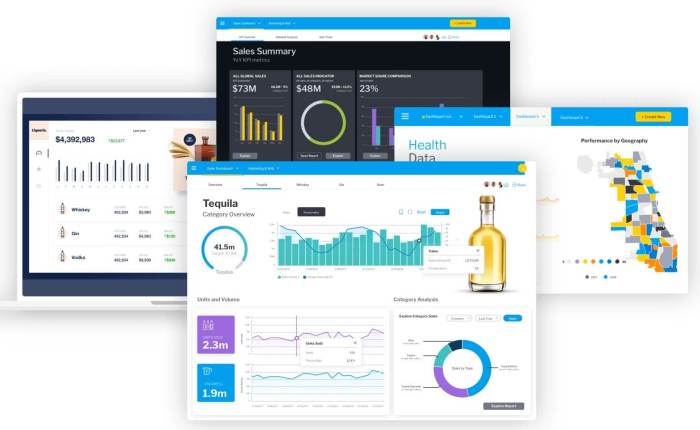
Harnessing the power of data for improved operational decision-making requires the right tools. Choosing the appropriate Business Intelligence (BI) platform and tools is crucial for effectively analyzing data and translating insights into actionable strategies. This section explores various BI technologies, their applications, and implementation strategies for enhancing operational efficiency.
Cloud-Based vs. On-Premise BI Platforms
The choice between cloud-based and on-premise BI platforms significantly impacts operational efficiency and cost. Cloud-based solutions, offered by providers like AWS, Azure, and Google Cloud, offer scalability, accessibility, and reduced infrastructure costs. They are ideal for businesses needing rapid deployment and flexible scaling to meet fluctuating data demands. On-premise solutions, on the other hand, provide greater control over data security and customization but require significant upfront investment in hardware and IT expertise.
They are more suitable for organizations with stringent security requirements or highly specialized data analysis needs. A key consideration is the level of IT support available internally; cloud solutions often require less internal IT expertise compared to on-premise systems.
Examples of BI Tools with Advanced Analytics Capabilities
Several BI tools offer advanced analytics features crucial for operational improvements. Tableau, for example, is known for its user-friendly interface and powerful visualization capabilities, allowing for easy exploration of operational data. Power BI, Microsoft’s offering, seamlessly integrates with other Microsoft products and offers robust data modeling and reporting features. Qlik Sense excels in associative data exploration, allowing users to uncover hidden relationships within their operational data.
These tools provide capabilities beyond basic reporting, including predictive modeling, machine learning algorithms, and what-if analysis, enabling data-driven forecasting and proactive decision-making.
Dashboard Design for Real-Time Operational Data
An effective operational dashboard displays real-time data in a clear, concise, and actionable manner. A well-designed dashboard should prioritize key performance indicators (KPIs) relevant to operational goals. For example, a manufacturing dashboard might display real-time production output, machine downtime, and inventory levels. Visualizations like gauges, charts, and maps are crucial for presenting complex data in an easily digestible format.
The dashboard should also incorporate interactive elements, allowing users to drill down into specific data points for deeper analysis. Color-coding can be used to highlight critical areas requiring immediate attention, such as production bottlenecks or quality control issues. Real-time data updates are essential, ensuring decision-makers have access to the most current information.
Implementing a New BI Tool in an Existing Workflow
Implementing a new BI tool requires a structured approach.
- Needs Assessment and Tool Selection: Clearly define operational challenges and objectives. Evaluate various BI tools based on features, scalability, cost, and integration capabilities with existing systems.
- Data Integration and Preparation: Identify and consolidate relevant data sources. Cleanse and transform data to ensure accuracy and consistency. This may involve ETL (Extract, Transform, Load) processes.
- Dashboard Design and Development: Design dashboards focusing on key operational KPIs. Develop reports and visualizations that provide actionable insights.
- User Training and Adoption: Provide comprehensive training to end-users on how to effectively utilize the new BI tool. Encourage user feedback to optimize the system.
- Monitoring and Optimization: Continuously monitor the system’s performance and make necessary adjustments to improve efficiency and accuracy. Regularly review and update dashboards to reflect evolving operational needs.
Improving Specific Operational Areas with BI

Business Intelligence (BI) isn’t just about collecting data; it’s about transforming raw information into actionable insights that drive operational excellence. By leveraging BI solutions, businesses can significantly optimize various operational areas, leading to increased efficiency, profitability, and customer satisfaction. This section will explore how BI specifically impacts supply chain management, customer service, manufacturing processes, and sales performance.
Supply Chain Optimization with BI
Effective supply chain management hinges on visibility and responsiveness. BI tools provide real-time dashboards displaying inventory levels, order fulfillment rates, and supplier performance. For instance, a retailer using BI might identify a consistent delay in shipments from a particular supplier, prompting proactive intervention to mitigate potential stockouts. Predictive analytics, a key component of many BI systems, can forecast demand fluctuations, enabling businesses to optimize inventory levels and avoid both overstocking and shortages.
This translates to reduced storage costs, minimized waste, and improved customer satisfaction through timely order fulfillment. Imagine a scenario where a clothing retailer, using BI-powered demand forecasting, anticipates a surge in demand for a specific item during a holiday season. They can adjust their production schedule, secure additional inventory, and even launch targeted marketing campaigns, maximizing sales and minimizing the risk of lost revenue.
Enhanced Customer Service and Retention through BI
BI plays a crucial role in understanding customer behavior and preferences. By analyzing customer data—including purchase history, website interactions, and customer service interactions—businesses can identify trends and patterns that inform improved customer service strategies. For example, a telecommunications company might use BI to identify customers at high risk of churning based on their usage patterns and recent support interactions.
This allows for proactive intervention, such as offering personalized retention deals or improved customer support, increasing customer loyalty and reducing churn rate. Further, analyzing customer feedback from surveys and social media can reveal areas needing improvement in products or services, leading to more customer-centric business decisions.
Smart business decisions hinge on robust business intelligence solutions, providing the data-driven insights needed for effective operational strategies. But how do you know if your BI investment is paying off? That’s where understanding the financial impact comes in, which is why checking out this guide on measuring the ROI of business intelligence investments in a company is crucial.
Ultimately, a clear ROI calculation helps justify continued investment in BI solutions that directly improve operational decision-making and bottom-line results.
Manufacturing Process Enhancement with BI
BI significantly enhances manufacturing efficiency by providing real-time visibility into production processes. Dashboards can track key performance indicators (KPIs) such as production output, defect rates, and machine downtime. Identifying bottlenecks and inefficiencies becomes straightforward, enabling manufacturers to optimize production schedules, reduce waste, and improve overall output. For example, a car manufacturer using BI might identify a specific assembly line consistently experiencing delays.
By analyzing the data, they can pinpoint the root cause, whether it’s a machine malfunction, insufficient workforce, or a flawed process, and implement corrective actions to streamline the production flow. This results in improved productivity, reduced manufacturing costs, and enhanced product quality.
Key Metrics for Measuring BI Impact on Sales Performance
Measuring the impact of BI on sales requires tracking relevant metrics. These include sales growth, conversion rates, average order value (AOV), and customer lifetime value (CLTV). For instance, a company implementing a BI-driven targeted marketing campaign can track the resulting increase in sales conversions and AOV to assess the campaign’s effectiveness. Similarly, analyzing CLTV can reveal the long-term profitability of different customer segments, guiding resource allocation and customer relationship management strategies.
Tracking these metrics provides quantifiable evidence of BI’s contribution to sales performance improvements. A software company, for example, might see a 15% increase in sales conversions after implementing a BI-driven lead scoring system that prioritizes high-potential leads. This demonstrably showcases the ROI of their BI investment.
Measuring the ROI of Operational BI Initiatives
Implementing Business Intelligence (BI) solutions requires a strategic approach, especially when considering the financial investment. Understanding and demonstrating the return on investment (ROI) is crucial for securing continued support and justifying future BI projects. This section Artikels a framework for measuring the ROI of operational BI initiatives, focusing on quantifiable metrics and practical reporting methods.
A robust ROI framework necessitates a clear understanding of both the costs and benefits associated with your BI project. Costs include software licenses, hardware, implementation services, training, and ongoing maintenance. Benefits, on the other hand, are harder to quantify but are ultimately where the value lies. They manifest as improvements in operational efficiency, cost reductions, revenue increases, and better decision-making.
Defining Key Performance Indicators (KPIs) for ROI Measurement
To accurately assess ROI, you must first identify and define relevant KPIs that directly reflect the impact of your BI initiatives on operational performance. These KPIs should align with your business objectives and be measurable using data collected through your BI system. Examples include reductions in production cycle times, improvements in inventory management, decreased customer churn, and increased sales conversion rates.
Choosing the right KPIs is crucial for accurately reflecting the value generated. For instance, tracking order fulfillment time before and after BI implementation directly shows the efficiency gains.
Quantifying the Impact on Operational Costs and Revenue, Business intelligence solutions for improving operational decision-making
Quantifying the impact of BI-driven decisions requires a methodical approach. For cost reduction, compare operational expenses before and after BI implementation. For example, if BI identified and eliminated redundant processes, calculate the savings in labor costs, material costs, or energy consumption. To quantify revenue increases, analyze sales data and correlate it with improved decision-making facilitated by BI.
For instance, if BI insights led to targeted marketing campaigns, calculate the increase in sales revenue generated from these campaigns. This requires establishing a baseline before BI implementation for effective comparison.
Reporting on BI Investment Value to Stakeholders
Stakeholders need clear, concise, and visually compelling reports to understand the value of BI investments. These reports should present both the costs and benefits in a clear and easily digestible manner. A simple table showing the reduction in operational costs and the increase in revenue, along with a calculation of the ROI, is highly effective. Visualizations such as charts and graphs can further enhance the understanding and impact of the report.
For example, a line graph illustrating the trend of reduced customer support calls post-BI implementation clearly demonstrates the efficiency improvements. Another example is a bar chart comparing the sales figures before and after the implementation of a BI-driven sales forecasting model.
Challenges in Accurately Measuring ROI and Mitigation Strategies
Accurately measuring the ROI of BI initiatives can be challenging. Attributing specific improvements solely to BI can be difficult, as other factors might contribute to the overall success. Furthermore, some benefits, such as improved employee morale or enhanced decision-making capabilities, are difficult to quantify directly. To mitigate these challenges, employ a control group for comparison, implement robust data tracking mechanisms, and utilize statistical analysis to isolate the impact of BI.
Conducting regular reviews and adjustments to the ROI measurement framework ensures its continued relevance and accuracy. For example, if a company implements a new BI system in one branch but not another, the performance of both branches can be compared to assess the impact of the system.
Case Studies
Real-world examples showcase the transformative power of Business Intelligence (BI) in optimizing operational efficiency across diverse industries. These case studies highlight how organizations leveraged data-driven insights to solve critical problems, streamline processes, and ultimately boost their bottom line. The following examples demonstrate the tangible benefits of strategic BI implementation.
Retail Inventory Optimization at a National Chain
Effective inventory management is crucial for retailers. Poor inventory control leads to lost sales due to stockouts and increased costs due to excess inventory. This case study focuses on a national retail chain that implemented a BI solution to optimize its inventory management.
- Problem: Inconsistent inventory levels across stores resulted in frequent stockouts of popular items and excessive holding costs for slow-moving products. This led to lost sales and reduced profitability.
- BI Solution: The retailer implemented a BI system that integrated data from its point-of-sale (POS) systems, warehouse management systems, and supplier databases. This allowed for real-time visibility into inventory levels, sales trends, and customer demand across all locations. Predictive analytics were used to forecast future demand and optimize inventory replenishment.
- Improvements: The BI system reduced stockouts by 15%, leading to a 10% increase in sales. Simultaneously, it reduced excess inventory by 20%, resulting in a 5% decrease in holding costs. This resulted in a significant improvement in overall profitability and customer satisfaction.
Illustrative Scenario: Imagine a popular summer dress consistently selling out in several stores. The BI system, by analyzing sales data and weather patterns, predicts a continued high demand. This allows the retailer to proactively increase orders from suppliers and redistribute inventory from stores with excess stock to those experiencing shortages, maximizing sales opportunities and preventing lost revenue.
Manufacturing Process Optimization in a Food Processing Plant
In the manufacturing sector, optimizing production processes is essential for efficiency and cost reduction. This case study examines a food processing plant that used BI to improve its production line efficiency.
- Problem: The food processing plant experienced frequent production line stoppages due to equipment malfunctions and material shortages. This resulted in lost production time and increased costs.
- BI Solution: The plant implemented a BI system that monitored real-time data from its production equipment, including machine performance, downtime, and material usage. This data was used to identify bottlenecks and areas for improvement in the production process. Predictive maintenance algorithms were also implemented to anticipate equipment failures.
- Improvements: The BI system reduced production line downtime by 25%, resulting in a 15% increase in production output. It also optimized material usage, reducing waste by 10%. This led to significant cost savings and improved overall operational efficiency.
Illustrative Scenario: The BI system detects a pattern of increased machine failures on a specific production line during peak operating hours. By analyzing the data, maintenance teams can proactively schedule repairs during off-peak hours, minimizing production disruptions and preventing costly downtime. This proactive approach significantly reduces the impact of equipment failures on overall production.
Healthcare Patient Flow Management in a Large Hospital
Efficient patient flow is crucial in healthcare settings to ensure timely treatment and reduce wait times. This case study details how a large hospital utilized BI to improve its patient flow management.
- Problem: The hospital experienced long wait times in the emergency department and operating rooms, leading to patient dissatisfaction and increased operational costs.
- BI Solution: The hospital implemented a BI system that integrated data from its electronic health records (EHR), scheduling systems, and patient tracking systems. This provided real-time visibility into patient flow, allowing staff to identify bottlenecks and optimize resource allocation.
- Improvements: The BI system reduced average wait times in the emergency department by 30% and in the operating rooms by 20%. It also improved resource utilization, leading to a 10% reduction in operational costs. This resulted in improved patient satisfaction and increased operational efficiency.
Illustrative Scenario: The BI system identifies a surge in emergency room patients during specific hours. Based on this data, hospital administrators can proactively adjust staffing levels and allocate resources to manage the increased patient volume, reducing wait times and ensuring timely treatment for all patients. This proactive approach minimizes disruptions and improves the overall patient experience.